How to Create a Realistic Data Analytics Strategy
Data analytics helps companies make better decisions based on real information.
That said, numbers show that 35% of people who lead data analytics teams have trouble showing how their work helps the company according to a 2024 report published by Gartner.
Also, 80% of attempts to control data properly fail unless there's an emergency that forces change. These numbers show why companies need a clear plan for using their data.
What Is a Realistic Data Analytics Strategy?
Every day, companies gather massive amounts of data. But after you do this, you need a clear plan to make this data useful.
When companies create detailed steps for handling their data, they can discover valuable insight, comply with data regulations, and guide data efforts to support meeting KPIs.
But realistically, also batch departments, sections, and projects to create an achievable timeline.
10 Steps to Building a Realistic Data Analytics Strategy
1. Define Business Objectives and Key Performance Indicators (KPIs)
Companies need to link their data initiatives directly to concrete business goals and establish clear protocols for data handling and results reporting.
With 56% of data leaders having increased their data analytics budget the fact is data governance and tracking ROI requires an extensive data analytics strategy.
Begin by identifying your company’s specific data objectives. Common goals can include things like:
- Making customers happier with your service
- Spending less money on daily operations
- Understanding what will happen in your market
- Getting products to customers faster
Track specific numbers that show progress, like how many customers stop using your service, how much money you make, or how long deliveries take.
A survey with 2024 statistics showed that business intelligence
This shows whether your data work actually helps the company.
2. Conduct a Data Audit
A data audit helps you spot gaps in your current datasets, making sure all necessary information is available for analysis.
The fact is that according to a recent survey by BARC companies consider data an asset according to 83% of data analytics professionals.
When you know exactly what data you have now, you can fix problems early and plan better for the future.
- Data Sources: List where you get data from inside your company (like sales systems) and outside (like market research)
- Data Quality: Check if your data is complete, correct, and makes sense
- Data Silos: Find places where important information sits alone and can't connect to other data.
You can use programs like Apache NiFi, Talend, and Informatica to check your data automatically.
3. Establish a Data Governance Framework
Create clear rules about data use to build trust and protect information. When everyone knows the rules about accessing and using data, you reduce problems with incorrect or misused information.
- Data Ownership: Decide who takes care of different types of data
- Compliance: Follow laws that deal data protection like GDPR, CCPA, and HIPAA to prevent expensive compliance fines and lawsuits
- Metadata Management: Use programs like Alation or Collibra to keep track of all your data
A strong governance framework prevents problems with privacy, security, and data quality
4. Build a Scalable Data Architecture Based on Use Cases
Building with future growth in mind allows your data systems to keep up with increasing needs.
Flexibility is important—designing a system that can handle new challenges without needing major changes will save time and resources as your company grows.
- Data Warehousing: Store organized data using programs like Snowflake, Google BigQuery, or Amazon Redshift
- Data Lakes: Keep raw, unorganized data in systems like Apache Hadoop or Azure Data Lake
- ETL/ELT Pipelines: Use tools like Apache Airflow, AWS Glue, or Fivetran to organize and move data efficiently
Adopting a hybrid approach—combining data warehouses and lakes—often delivers the best results for diverse analytics needs.
5. Choose the Right Analytics Tools
Choosing the right tools means finding the balance between functionality and ease of use. It’s important to pick tools that meet your current needs but also have room to expand as your data demands grow in the future.
- Data Visualization: Tableau, Power BI, Looker
- Business Intelligence (BI): Qlik Sense, Domo, MicroStrategy
- Machine Learning Platforms: Databricks, TensorFlow, AWS SageMaker
- Real-Time Analytics: Apache Kafka, Apache Flink, Spark Streaming
Ensure that selected tools integrate seamlessly with your existing tech stack and support future expansion.
6. Develop a Data Integration Strategy
Without proper connectivity, data remains isolated and cannot be fully used for decision-making. A solid strategy for linking systems ensures all platforms work together, giving you a complete view of your data. This access to all your information leads to more precise, real-time insights, helping you make better decisions.
- APIs and Middleware: Use platforms like MuleSoft or Dell Boomi for seamless connectivity.
- Data Fabric: Employ solutions such as IBM Cloud Pak for Data to enable unified access across distributed environments.
- Streaming Platforms: Integrate real-time data using Confluent or Azure Stream Analytics.
Integration ensures a unified view of enterprise data, enabling better insights.
7. Empower Data Teams with Skills and Resources
Training employees not only increases productivity but also boosts morale and retention. By investing in their development, you build a culture of innovation that fuels business growth. Hands-on experience with modern tools sharpens their ability to tackle complex challenges.
- Training Programs: Offer certifications in Python, R, SQL, and AI/ML frameworks.
- Collaborative Environments: Provide access to Jupyter Notebooks, Databricks Workspaces, or Google Colab.
- Cross-Functional Collaboration: Foster partnerships between IT, data scientists, and business stakeholders.
Equipping teams with the right skills accelerates analytics adoption and drives impactful outcomes.
8. Start with Pilot Projects
Starting with small-scale projects allows you to identify challenges early and adjust before expanding. By learning from these initial efforts, you can design more effective strategies for future projects. Success in pilot projects shows the potential value of analytics and encourages broader use across the company.
- Test your data pipeline’s efficiency
- Validate the ROI of analytics initiatives
- Refine your approach based on real-world feedback
Once proven, scale successful projects across other departments or business units.
9. Implement Real-Time Analytics
Real-time analytics gives instant insights, helping businesses stay ahead of competitors. Continuously processing live data allows for quicker, more informed decisions that have a direct impact. The ability to respond quickly makes all the difference between success and missed opportunities.
- Fraud detection
- Predictive maintenance
- Personalized marketing
Technologies such as Apache Kafka, AWS Kinesis, and Google Cloud Dataflow enable processing and analyzing streaming data in real-time, driving faster decisions.
10. Monitor, Optimize, and Evolve
Regular monitoring helps ensure that your analytics stay on track with shifting goals and market changes. Optimization not only boosts efficiency but also uncovers fresh growth opportunities. By staying flexible and adjusting as needed, your analytics stay relevant and competitive over time.
- Dashboards: Create real-time views of analytics performance using Tableau or Power BI.
- Automation: Deploy tools like Apache Beam or MLflow for automated model monitoring.
- Feedback Loops: Regularly collect stakeholder input to align analytics initiatives with business needs.
Driving Financial Outcomes Through Analytics
Enterprises invest in data analytics not just to generate insights but to achieve measurable financial gains. Here’s how:
Cost Optimization
By using data to identify where money is being wasted, businesses can make better decisions about where to cut costs. Whether it’s optimizing supply chains or improving workflow efficiency, analytics helps find areas for improvement. Reducing waste in operations can lead to significant savings and more efficient use of resources.
Revenue Growth
Analytics doesn’t just help predict what customers want—it also helps businesses figure out the best ways to meet those needs. By analyzing past behavior and trends, companies can spot patterns that lead to new revenue streams. A deeper understanding of customer preferences leads to more accurate pricing and better-targeted promotions.
Risk Mitigation
Advanced analytics allows businesses to detect patterns of risky behavior before they cause damage. By analyzing historical data, companies can identify trends that lead to potential security or financial issues. This proactive approach helps minimize damage and protects both assets and reputation.
Why Work With PowerBI and Tableau Professionals?
Creating a realistic and effective data analytics strategy can be complex from a technical standpoint. And realistically speaking, it needs to look at your business goals, technical capabilities, and team’s readiness.
Creating a data analytics strategy curated for your business working with PowerBi and Tableau professionals can get your business started with a system that management can rely on through and through.
And after this, you can maintain this with the use of your existing technicians with minimal technical training.
Sound promising? Reach out for a free consultation call with our certified data analysts!
Stay ahead with our IT Insights
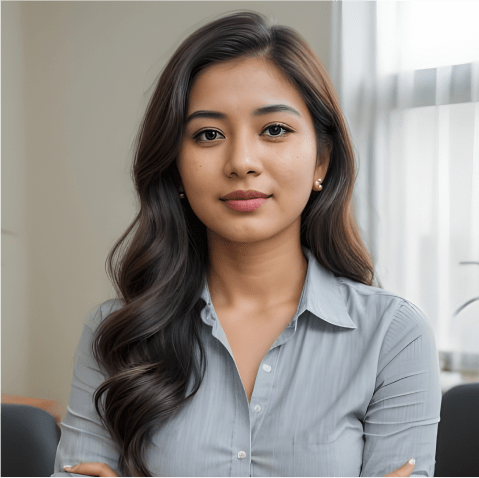
Discover Your AI Agent Now!
An AI Agent Saved a SaaS Company 40 Hours in a Week!