Evolution of Generative AI: Shaping Tech Solutions
What is Generative AI?
Generative AI refers to a subset of artificial intelligence focused on creating new data similar to existing data. Unlike traditional AI systems that recognize patterns or make decisions, generative AI can produce content, such as text, images, music, or even complex models.

How Does Generative AI Work?
Generative AI relies on advanced models to create data, including Generative Adversarial Networks (GANs), Variational Autoencoders (VAEs), and Neural Language Models (NLMs). These systems learn from data to generate outputs that mimic human creativity.
GANs, VAEs, NLMs
- GANs: These involve two models—a generator and a discriminator—that work together to create realistic outputs.
- VAEs: These models encode data into a compressed format and decode it to generate new variations.
- NLMs: Examples include GPT, which generates human-like text by predicting words based on context.
Gen AI Vs. Discriminative AI: Two Different Things
Generative AI and Discriminative AI serve different purposes. While discriminative models classify data and make decisions, generative models focus on creating new data.
Understanding this distinction helps in choosing the right approach for specific tasks.
- Generative AI creates content, while discriminative AI analyzes it.
- Each serves unique applications in AI-driven projects.
- Choosing the wrong model can lead to inefficiencies.
The Evolution of Generative AI: A Timeline
Recurrent Neural Networks (RNNs) and LSTM Networks - 1980s
RNNs and Long Short-Term Memory (LSTM) networks were the first steps in building AI systems capable of processing sequences, such as text and speech.
- These systems paved the way for natural language processing.
- They introduced the ability to remember past inputs for better predictions.
- Early models were limited by computational power and data availability.
Generative Adversarial Networks (GANs) - 2014
GANs revolutionized generative AI by enabling systems to produce hyper-realistic images, videos, and other content. This innovation significantly impacted industries like design, entertainment, and e-commerce.
- GANs involve two models working against each other, refining outputs iteratively.
- They require substantial computational resources and expertise.
- The introduction of GANs marked a turning point for creative AI applications.
Generative AI in the 2020s
Generative AI went from experimental to essential in the 2020s, thanks to rapid improvements in computing and groundbreaking models like ChatGPT, Gemini, and Llama.
These technologies are changing industries by supercharging both productivity and creativity, bringing AI into everyday tasks in ways we couldn’t have imagined before.
ChatGPT
ChatGPT has transformed how businesses interact with customers and manage content. This makes it more than just a chatbot—it’s more like a tool capable of creating personalized marketing campaigns, drafting complex reports in seconds, and automating processes across departments.
Understanding and mimicking human conversation, helps companies save time and connect more authentically with their audiences.
- Use guardrails to prevent biased or harmful content.
- Ensure APIs and deployment environments are secure.
- Regularly update the model to reflect the latest data and trends.
Gemini by Google (Formerly Bard)
Gemini has redefined contextual understanding, making it a game-changer for industry-specific solutions.
Whether it’s helping doctors diagnose diseases faster or enabling architects to create sustainable designs, Gemini’s ability to process nuanced information has set a new standard for AI tools. It’s particularly valuable in handling complex, specialized tasks that demand deep insights.
- Competes directly with other advanced AI systems.
- Leverages Google’s vast data resources for unique insights.
- Highlights the need for differentiation in a crowded market.
Llama from Meta
Llama has made collaborative innovation more accessible than ever. By prioritizing open-source principles, it empowers researchers and developers to push the boundaries of AI.
This generative AI framework creates breakthroughs in areas like climate modeling, educational tools, and social impact initiatives. However, it also raises new questions about ethical data use and licensing, making it a catalyst for important industry conversations.
Top Applications of Generative AI
Generative AI in SaaS
SaaS platforms now harness generative AI to revolutionize how businesses operate. By automating repetitive processes, improving customer interactions, and crafting tailored solutions, generative AI empowers companies to save time and innovate faster. It enhances the adaptability of SaaS tools, making them smarter and more intuitive for users.
- Simplifies repetitive tasks for businesses.
- Improves user experience through customization.
- Supports scalable solutions for various industries.
Use of Generative AI in Healthcare
Generative AI is transforming healthcare by accelerating drug discovery, refining medical imaging, and enhancing patient diagnosis accuracy.
It cuts down the time and cost involved in clinical trials and provides tools that empower doctors with precise, data-driven insights. This technology also opens doors for personalized treatment plans tailored to individual patient needs.
- Reduces time for drug development and testing.
- Enhances the accuracy of diagnostic tools.
- Raises ethical concerns around data privacy.
Content Creation
Generative AI is redefining content creation by enabling the rapid production of articles, videos, images, and audio.
Creators can scale their projects with ease, using AI to brainstorm ideas, draft high-quality material, and automate repetitive tasks. While AI speeds up the creative process, human oversight ensures the content remains accurate and unbiased.
Generative AI in Marketing and Scenario Creation
Marketing has embraced generative AI to craft campaigns that feel personal, analyze customer behavior, and predict future market trends.
AI helps create engaging advertisements, simulate potential scenarios, and uncover opportunities that drive success. It empowers marketers to make data-informed decisions and stay ahead in competitive landscapes.
Gen AI in Media and Entertainment
Generative AI is reshaping media and entertainment by streamlining production processes and offering new creative possibilities.
From generating realistic special effects to composing original music, AI tools are pushing the boundaries of what creators can achieve. While boosting efficiency, these innovations also prompt discussions around ethical use and intellectual property.
- Enhances creative processes with innovative tools.
- Reduces production costs and timelines.
- Raises ethical concerns, such as copyright issues.
Advantages and Challenges of Generative AI
Advantages of Generative AI
- Less Repetitive Tasks: Speeds up processes and reduces operational costs. By automating repetitive tasks using Gen AI, businesses can save time and allocate resources more efficiently, leading to significant cost savings.
- Has a Broader Knowledge Base: Unlocks new creative possibilities in various fields. From designing unique marketing campaigns to generating original artwork, generative AI enables professionals to think beyond traditional boundaries.
- Allows Personalization at Scale: For example, it can tailor online shopping experiences by suggesting products based on user preferences, enhancing customer satisfaction.
- Enhances decision-making with data-driven insights. AI models can analyze vast amounts of data quickly, helping industries like finance and healthcare make better, faster decisions.
- Improves accessibility through advanced language translation. Tools like ChatGPT can bridge communication gaps, making information available to people in multiple languages.
- Empowers education with interactive learning tools. AI-driven applications can adapt to individual learning paces, offering personalized tutoring experiences for students.
- Enables rapid prototyping in industries like architecture and engineering. Generative models can create multiple design iterations in hours, saving weeks of work.
- Boosts scientific research with data analysis capabilities. For instance, AI is used to accelerate drug discovery by identifying potential compounds in a fraction of the usual time.
Challenges of Generative AI
- Misuse and Misinformation: Generative AI can create realistic fake news, deepfakes, or misleading content, making it harder to trust digital information.
- Depends heavily on high-quality data: Without accurate and diverse datasets, the outputs can reflect biases or inaccuracies, limiting the AI’s effectiveness.
- Requires significant computational resources: High energy consumption and expensive hardware can limit accessibility for smaller organizations or researchers.
- Poses ethical dilemmas: For example, determining who owns AI-generated content remains a complex issue.
- Increases job displacement: Automation of tasks like content writing or customer service could lead to workforce reductions.
- Challenges regulatory frameworks: Governments struggle to create policies that keep pace with the evolving capabilities of generative AI.
- Privacy Concerns with Data Usage: AI often relies on large-scale personal data, increasing the risk of breaches and misuse.
- Struggles with interpretability and transparency: Understanding how AI models arrive at their outputs can be difficult, making it hard to trust or verify their results.
The Future of Generative AI in Modern Tech Solutions
More Demand for Industry and Domain-Specific AI Models
Modern AI platforms allow enterprises to fine-tune pre-trained models to align with niche use cases.
More importantly, they support multi-modal frameworks capable of processing varied data types—text, images, and even structured data—enabling cross-functional workflows and deeper insights.
How so? These models leverage transfer learning techniques to optimize performance for specific domains, offering scalability while maintaining operational consistency across diverse applications.
Shifting Towards More Energy Conserving Techniques in Gen AI
Transitioning to more energy-conscious models involves optimizing algorithms and adopting hardware accelerators designed for specific workloads. These approaches reduce computational overhead and improve processing efficiency.
Additionally, edge computing frameworks are increasingly utilized to process data locally, minimizing reliance on energy-intensive cloud systems. By consolidating these techniques, enterprises can achieve sustainable AI deployment without compromising model performance or scalability, ensuring a balance between innovation and environmental responsibility. What this will do is:
- Reduces the environmental impact of AI development.
- Cuts operational costs for businesses.
- Encourages innovation in hardware and algorithms.
More Integration of Generative AI With Equipment and Robotic Systems
Since robotic systems and equipment often operate with distinct protocols, aligning generative AI capabilities across these systems can be complex due to varied operational frameworks. By incorporating generative AI, you can synchronize task execution and refine autonomous operations.
More importantly, generative AI can predict system behaviors and optimize robotic workflows, reducing downtime and improving precision in real-world applications
Using AI with robotics can revolutionize industries like manufacturing, logistics, and healthcare.
Use of AI-Generated Data to Create Realistic Models and Solve Absence of Existing Information
Since generative AI relies on vast datasets, addressing gaps in existing information can be challenging due to the variability of available data. By using AI-generated synthetic datasets, models can simulate realistic scenarios that fill these voids effectively.
AI can and will be used more to fill gaps in data, creating realistic models for simulations and decision-making.
- Enhances planning and forecasting capabilities.
- Supports innovation in fields with limited data.
- Raises concerns about the validity of synthetic data.
How Entrans Can Help You Use Generative AI
Entrans offers expertise in generative AI, helping businesses unlock its potential while addressing challenges.
Generative AI can be used to improve your existing framework, automating even the most mundane tasks.
Generative AI is a game-changer, and staying ahead requires continuous learning, innovation, and ethical implementation. By embracing this technology thoughtfully, businesses can shape the future of their industries.
Want to know more about this? Reach out to our AI team for a free call!
Stay ahead with our IT Insights
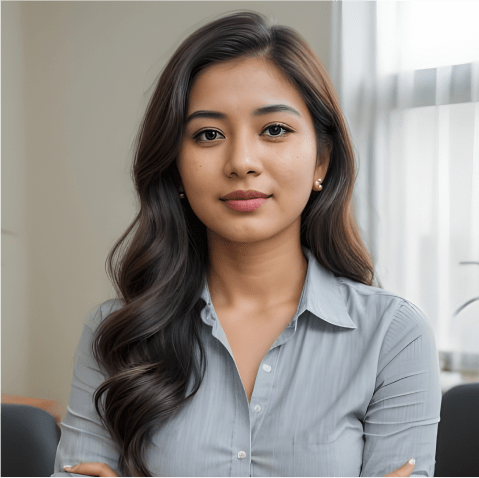
Discover Your AI Agent Now!
An AI Agent Saved a SaaS Company 40 Hours in a Week!