What is the First Step of the Data Analytics Process?
Data is everywhere, but not all data leads to valuable insights. Picture this: a retail company notices declining sales and wants to understand why. They collect massive amounts of data—customer purchases, website traffic, social media engagement—but without a clear starting point, they risk drowning in information without actionable insights. This is where the first step of the data analytics process comes into play: defining the problem.
In this blog, we'll explore why defining the problem is crucial, how to do it effectively, and common mistakes to avoid. By the end, you’ll have a structured approach to kickstarting a successful data analytics project.
What is the First Step of the Data Analytics Process?
The first and most crucial step in data analytics is defining the problem or research question. Before diving into data collection, businesses must have a clear understanding of what they aim to achieve. Without this clarity, the analysis may become directionless, leading to misleading insights.
For instance, in healthcare, a hospital analyzing patient data should first define whether they are looking to reduce readmission rates, improve patient satisfaction, or optimize resource allocation. Each of these goals requires different data and analytical approaches.
Why is Defining the Problem So Important?
Ensures Focused Data Collection
A well-defined problem helps in collecting only relevant data, reducing noise and increasing efficiency. For example, if an e-commerce company wants to understand high cart abandonment rates, they should focus on behavioral data rather than overall sales trends.
Saves Time and Resources
Organizations can save significant time and costs by focusing on a specific, well-defined question. According to a Gartner report, 85% of data science projects fail due to poorly framed problem statements.
Leads to Actionable Insights
When the problem is clearly defined, the insights generated are actionable and impactful. A telecom company, for example, analyzing churn rates without a clear question may find general trends but struggle to implement targeted retention strategies.
How to Define the Right Problem Statement?
1. Understand Business Objectives
Before defining the problem, align with key stakeholders to understand what success looks like. A finance team may want insights into cost reductions, while marketing may prioritize customer engagement.
2. Use the SMART Framework
A well-defined problem statement should be:
- Specific: Clearly state the issue (e.g., "Why are first-time users abandoning our app after one session?")
- Measurable: Ensure it can be quantified (e.g., "Decrease churn rate by 10% in six months.")
- Achievable: Set realistic expectations.
- Relevant: Align with business goals.
- Time-bound: Define a timeframe for analysis.
3. Formulate a Hypothesis
Develop a hypothesis that can be tested with data. For example, a retail store might hypothesize that "Customers abandon their carts due to high shipping costs."
Common Mistakes in Defining the Problem
Vague or Broad Problem Statements
A problem like "Why are sales dropping?" is too broad. Instead, it should be more precise: "Why have sales declined by 15% in the Northeast region in Q1?"
Ignoring Stakeholder Input
Failing to involve key decision-makers can lead to misaligned goals and wasted efforts.
Confusing Symptoms with Problems
If customer complaints about long wait times are increasing, the real issue might be understaffing rather than slow service.
What Comes After Defining the Problem?
Once the problem is defined, the next steps in the data analytics process include:
- Data Collection: Gathering relevant data from internal and external sources, such as company databases, market reports, surveys, and third-party data providers. This step ensures that analysts have enough information to explore trends and patterns that relate to the defined problem.
- Data Cleaning & Preparation: Before analysis, raw data must be cleaned and organized to remove inconsistencies, errors, and duplicates. This step includes formatting datasets, handling missing values, and ensuring that all variables are properly structured. A well-prepared dataset improves the accuracy of the analysis and prevents misleading insights.
- Data Analysis: Once the data is cleaned, various statistical, analytical, or machine learning models are applied to uncover insights. Techniques such as regression analysis, clustering, and predictive modeling help businesses make sense of data, identify trends, and forecast future patterns based on historical information.
- Interpretation & Reporting: The results of the analysis must be translated into meaningful insights that decision-makers can understand. Data visualization techniques, including charts, graphs, and dashboards, help convey complex findings in an accessible manner. Reports should highlight key takeaways and recommendations aligned with the business objective.
- Decision Making & Implementation: Finally, the insights derived from data analysis are used to make informed business decisions. Whether it's optimizing marketing campaigns, improving customer experience, or streamlining operations, companies must take actionable steps based on data-driven recommendations to achieve their goals.
Conclusion
Defining the problem is the foundation of any successful data analytics project. Without a well-structured problem statement, even the most advanced analytical techniques may fail to deliver meaningful insights. Whether you’re a business leader, data analyst, or researcher, mastering this first step will set you on the path to making informed, data-driven decisions.
Stay ahead with our IT Insights
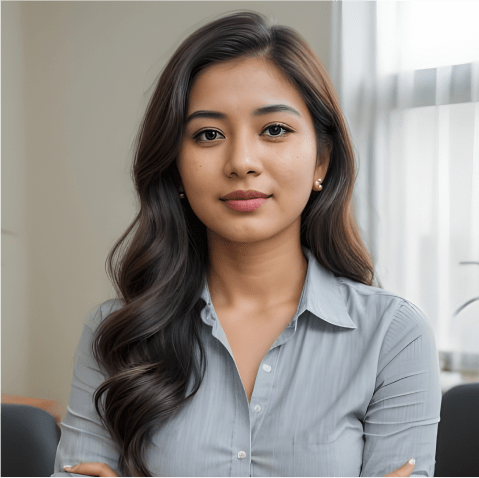
Discover Your AI Agent Now!
An AI Agent Saved a SaaS Company 40 Hours in a Week!