From Vision to Reality: How Generative AI is Shaping Healthcare Technologies
Generative AI is transforming healthcare by introducing innovative ways to process data, improve patient outcomes, and speed up processes formerly riddled with bottlenecks. Here’s how:
Key Takeaways: Understanding Generative AI in Healthcare
- Generative AI in healthcare uses advanced algorithms to simulate human-like creativity, making it a valuable tool in medical research and clinical settings.
- By generating insights from vast amounts of data, it allows healthcare providers to make informed decisions faster.
- That said use of this tech requires careful consideration of privacy, security, and the ethical use of patient information.
Real-World Use Cases of Generative AI in Healthcare
1. Automation of Administrative Tasks
Generative AI can handle time-consuming administrative tasks, freeing up valuable time for healthcare professionals to focus on patient care.
Automating repetitive processes reduces errors and improves efficiency in managing operational workflows. This transformation adds faster service delivery and enhances the patient experience. However, adopting AI in these tasks requires careful alignment with legal and privacy regulations to protect sensitive data.
I. Filling Insurance Claims and Billing
Generative AI accelerates insurance claims by analyzing and populating forms accurately. It reduces manual errors and ensures faster reimbursement processes, which benefits both providers and patients.
II. Documentation and Record-Keeping
AI systems can create and manage detailed records efficiently, ensuring that healthcare professionals always have access to accurate patient information. This reduces the risk of miscommunication and enhances continuity of care.
III. HIPAA and Other Compliance Requirements
Generative AI must adhere to strict compliance standards like HIPAA to protect patient data. It is essential to regularly audit AI systems to ensure they meet all legal and ethical guidelines.
2. Development of Pharmaceuticals
Generative AI is revolutionizing drug discovery by speeding up the research process and making it more cost-effective. It helps scientists identify promising compounds, predict their effects, and design safer drugs.
This reduces the overall timeline for getting new treatments to market. However, integrating AI into pharmaceutical development requires rigorous testing and validation to maintain safety standards.
- Quicker Compound Generation AI can analyze millions of molecular structures to suggest viable drug candidates in a fraction of the time it would take humans. This accelerates the initial stages of drug development significantly.
- Predicting Drug Responses and Reactions: By simulating how drugs interact with the human body, AI can forecast their effectiveness and potential side effects. This minimizes risks in clinical trials and enhances patient safety.
- More Accurate Prediction of Adverse Effects: AI systems can predict adverse effects early in the drug development process, reducing the likelihood of complications during trials. This ensures that only the safest drugs move forward in the pipeline.
3. Medical Data Analysis
Generative AI can analyze complex medical data to uncover patterns and trends that are difficult for humans to detect. It enhances decision-making and supports more accurate diagnoses. However, ensuring data quality and interoperability is critical to maximize its benefits.
- Better Trend Analysis: AI can identify emerging trends in patient health data, helping healthcare providers anticipate outbreaks or chronic disease patterns. This proactive approach improves preventive care.
- Data Processing and Integration: Generative AI processes vast datasets from multiple sources, ensuring seamless data integration. This creates a comprehensive view of patient health, enabling more effective treatment plans.
- Creation of More Accurate and Up-to-date Data pipelines: AI helps build reliable data pipelines that keep information current, improving the accuracy of medical research and care delivery. Maintaining these pipelines requires robust data governance.
- Natural Language Processing: NLP enables AI to interpret and analyze medical text, such as physician notes and research papers. This enhances the accessibility and usability of unstructured data in healthcare.
4. Medical Imaging for Visual Depiction and Spotting Conditions
Generative AI enhances medical imaging by improving the clarity of images and identifying abnormalities with high accuracy. These advancements aid in early diagnosis and better treatment planning. However, maintaining data integrity and addressing potential biases in imaging algorithms are crucial.
I. Image Enhancement and Noise Reduction
AI improves the quality of medical images by removing noise and enhancing details, enabling more accurate diagnoses. This is especially valuable in cases where imaging conditions are less than ideal.
II. Spotting Cancerous Growths Through Image Recognition Software
AI-powered tools can detect cancerous growths in their early stages, increasing the chances of successful treatment. These systems must be continually trained to improve their accuracy.
III. Data Augmentation
Generative AI creates synthetic imaging data to train models, ensuring they perform well across diverse scenarios. This reduces the need for extensive real-world imaging datasets.
5. Creating Synthetic Data for Medical Research
Synthetic data generated by AI can fill gaps in existing datasets, enabling better research and training for AI systems. It is particularly valuable in protecting patient privacy while maintaining data utility. Ensuring synthetic data accurately represents real-world scenarios is essential.
I. Creates More Diverse Sample Groups
Generative AI produces synthetic data to address gaps in diversity, improving the inclusivity of medical research and treatments.
II. Easier Compliance with HIPAA and Data Privacy Laws
By using synthetic data instead of real patient information, healthcare organizations can comply with privacy laws while still gaining insights from their data.
III. Realistic Data Creation and Validation
AI creates realistic datasets for testing and validating new algorithms, ensuring their effectiveness before deployment in real-world settings.
6. Personalized Treatment and Medical Approaches Using Gen AI
Generative AI supports personalized medicine by analyzing genetic, environmental, and lifestyle data. It enables healthcare providers to design treatments tailored to individual patients. However, achieving this level of personalization requires high-quality data and robust ethical considerations.
- Data Analysis and Spotting Unique Genetic Markers: AI identifies genetic markers that may influence disease risk or treatment response, paving the way for targeted therapies.
- Better Pharmacogenomic Information: By analyzing genetic data, AI helps predict how patients will respond to medications, reducing the risk of adverse reactions and improving outcomes.
- Insurance and Reimbursement: Generative AI assists in creating accurate patient profiles for insurance purposes, ensuring that treatment plans align with coverage and reimbursement policies.
Benefits of Generative AI in Healthcare
1. Quicker Timelines in Processing Medical Results and Paperwork
Generative AI reduces turnaround times for medical paperwork and test results, allowing healthcare providers to focus on patient care. This speeds up treatment decisions and improves efficiency.
What generative AI does is allow data standardization and automated report generation, simplifying workflows and reducing manual intervention.
How so? Well, It uses advanced natural language models to extract, analyze, and structure data efficiently.
2. Less Human Error in Medical Data Entry
Since healthcare platforms rely on accurate data entry, reducing inconsistencies across systems can be challenging due to manual processes. With generative AI, data entry workflows across platforms can become far more consistent and reliable.
By automating data entry, AI minimizes mistakes that could lead to misdiagnoses or delays. Accurate records enhance patient safety and trust.
3. Higher Chances of Spotting Potential Terminal Conditions at the Right Stage
Spotting terminal conditions at the right stage can be hard due to inconsistent data analysis. With generative AI, diagnostic processes across platforms can become more accurate and timely.
More importantly, generative AI employs predictive modeling and anomaly detection to analyze complex datasets, improving the precision of early-stage condition identification.
AI’s ability to analyze data rapidly increases the chances of detecting terminal conditions early. Early detection leads to more effective treatment and better outcomes.
4. Less Risky Drug Testing on Both Animals and Humans Through Simulations
Since drug testing involves varied systems and high stakes, conducting trials on animals and humans can be complex due to ethical and logistical challenges. With generative AI, simulations for testing can significantly reduce these risks.
Aside from this, generative AI uses advanced modeling techniques to replicate biological responses, creating virtual scenarios that predict drug efficacy and safety with greater accuracy.
5. Can Help Doctors Handle Invasive Procedures Better by Creating Accurate Imagery
A lot of the time medical procedures require precise visualization, and handling invasive surgeries can be challenging due to varying imaging technologies. With generative AI, imaging across different systems can become more accurate and consistent.
AI-generated imagery aids doctors during invasive procedures by providing precise visualizations. Gen AI processes patient data to generate 3D models and simulations, giving surgeons a clearer view of complex anatomy. This reduces reliance on traditional imaging systems and supports better outcomes
6. Better Processing of Large Quantities of Data When Used With ML
Everyday, healthcare systems deal with massive amounts of information, and processing this data can be slow and error-prone. With generative AI, handling large datasets becomes faster and more precise.
Generative AI combined with machine learning processes massive datasets quickly and accurately. This improves decision-making and research capabilities.
Challenges in Using Generative AI in Healthcare
1. Bias Mitigation
Healthcare data comes from diverse sources, and managing biases in AI models can be a challenge due to varying data quality. With generative AI, dealing these biases becomes more feasible.
Also, advanced algorithms can be used to identify and correct biased patterns in training datasets, improving model fairness.
AI systems must be designed to avoid biases that could impact patient care. Regular audits and diverse datasets are crucial to addressing this issue.
2. Ethical Guidelines
Using generative AI in healthcare brings ethical challenges, especially around transparency and patient data security. With a generative AI system, you can make sure that ethical guidelines around data handling and decision-making are consistently applied across all AI models.
What’s more, AI frameworks can be configured to monitor data usage and maintain transparency, making it easier to identify biases and track compliance with ethical standards.
3. Complicates Validation and Compliance
With AI models generating clinical insights, confirming their accuracy and aligning with medical standards becomes a challenge. A lot of countries follow HIPAA and GDPR guidelines which makes the potential issue as well!
But to deal with this, AI systems can be standardized to follow regulatory frameworks, ensuring that outputs meet legal requirements across platform
4. Scalability and Use With Existing Analog and Legacy Systems
Generative AI in healthcare can be challenging when working with existing analog and legacy systems. Adapting AI models to function alongside older technologies can create inconsistencies.
For anyone to handle this, AI systems need to have compatible frameworks using API and SDK or Network access gateways, this makes handling the process with info stored on legacy systems and applications a lot easier for cloud-based AI models.
5. Frequent Training of Medical Personnel to Handle Generative AI Processes
Using Generative AI and AI systems in healthcare often results in complex medical insights. To deal with this, healthcare workers must stay updated on how to interpret and act on these results.
By using standardized frameworks across systems, medical staff can quickly adapt to new AI tools.
6. Hard to Scale and Source AI Knowledgeable Resources
As AI models become more complex, sourcing qualified experts to handle and maintain them becomes difficult.
Finding skilled professionals to develop and maintain AI systems can be difficult. And to combat this, Investment in education and partnerships is needed to address this gap.
How Entrans Facilitates Generative AI Deployment in Healthcare
Entrans simplifies the deployment of generative AI by offering tailored solutions that align with healthcare needs. Their expertise ensures seamless integration, improved workflows, and adherence to privacy and ethical standards.
Entrans helps healthcare providers maximize the benefits of generative AI while addressing challenges effectively also improving the RCM process and automating your data engineering pipelines.
Want to know how we can help? Reach out for a free consultation!
Stay ahead with our IT Insights
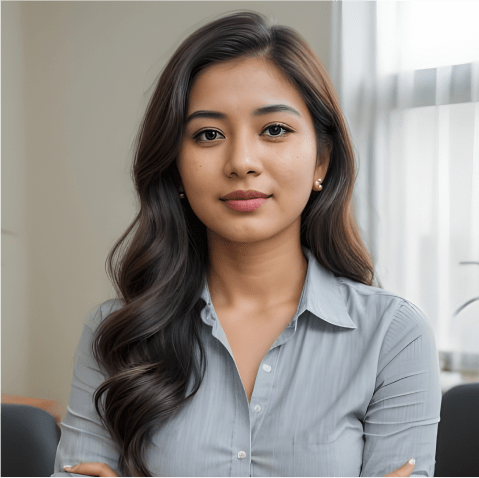
Discover Your AI Agent Now!
An AI Agent Saved a SaaS Company 40 Hours in a Week!