Supply Chain Predictive Analytics: Key Benefits and Use Cases
Supply chain predictive analytics leverages advanced data analysis techniques, including machine learning and statistical modeling, to forecast future trends and disruptions in supply chains.
By analyzing historical data and external factors, businesses can anticipate demand, optimize inventory, and mitigate risks before they occur. This proactive approach transforms traditional supply chain management into a data-driven, efficient process that enhances decision-making and reduces costs.
As predictive analytics evolves with AI and IoT, its role in supply chain optimization continues to grow, providing companies with the tools to stay ahead in today’s competitive market.
What is Supply Chain Predictive Analytics?
Definition and Key Concepts
At its core, supply chain predictive analytics is to analyze historical data, statistical models, and machine-learning algorithms in order to predict supply chain happenings in the future. Such incidents might include demand-spike forecasting, inventory-level optimization, disruption predictions, and transportation route optimization. Supply chain predictive analytics goes beyond descriptive analysis by delivering prescriptive insights for future decision-making.
The Role of Predictive Analytics in Supply Chain Management
Predictive analytics in supply chain management has made a tremendous difference in the modern supply chains because it enhances visibility and empowers proactive decision making. An example will be predicting low inventory levels by the supply chain manager or the time when demand for a particular product goes high. With predictive analytics in supply chain, companies can use data from multiple channels for optimization and prevention before the issue boils over into a crisis.
How Predictive Analytics Differs from Traditional Analytics
Predictive analytics marks a major shift from traditional analytics, which emphasizes describing past occurrences, such as, "What happened?" whereas supply chain predictive analytics is really more forward-looking in asking, "What is likely to happen?" This future-mindedness allows them to develop plans rather than react to trends that have happened in the past, thus giving them an upper hand in today's fast-paced markets.
The Importance of Predictive Analytics in Supply Chains
Enhancing Supply Chain Visibility
As global supply chains heat up, it can be really tough to get complete visibility into them. As supply chain predictive analytics takes everything data-capturing and reaches beyond supply chain limits, they could make businesses become even smarter in terms of visibility and, therefore, give better decisions possible. However, these can also make it easier to predict possible future conditions to avoid any bottlenecks, paving a smoother new path for operations.
Proactive Decision-Making and Risk Management
Predictive analytics allow firms to make the shift from reactive to proactive decision-making. Disruptions such as supplier delays and transportation issues can be anticipated, allowing for risk mitigation before supply chains are affected. This becomes all the more crucial when disruptions arise due to natural disasters or geopolitical events, for which companies prepare with contingency plans.
Optimizing Inventory and Demand Forecasting
One of the greatest challenges in the field of supply chain management is balancing supply with demand. Predictive analytic forecasting, in fact, utilizes historical sales data, seasonal trends, and market conditions to project future needs more effectively. Thus, it helps the companies optimize their inventory levels and reduce stockouts while avoiding cases of overstocking. Consequently, they are guaranteed to have the right products available at the right time.
How Supply Chain Predictive Analytics Works
Data Collection and Integration
Gathering relevant data is the first step of supply chain predictive analytics. This data can be from different sources like sales history, market trends, customer behavior, and external factors, which cover anything from weather patterns to geopolitical shifts. The successful integration of this disparate data is necessary for accurate prediction.
Statistical Modeling and Regression Analysis
Statistical models like regression analysis are employed to detect relationships among variables. For example, a company may use regression analysis to understand how sales data correlates with pricing, weather, or regional economic conditions, thereby assisting the company in forecasting future sales and demand.
Machine Learning and AI in Predictive Analytics
Over time, such machine learning algorithms become fairly accurate in predictions by learning from some past data and modifying the models. AI-enabled tools are able to identify extremely complex patterns that are beyond human reach to spot, thus gradually improving an organization's predictive capabilities as it gradually feeds more data in.
Key Use Cases of Predictive Analytics in Supply Chain Management
Demand Forecasting and Planning
To forecast future demand, estimate the amount of inventory required at a specific point in time, thus reducing storage expenses and preventing stockouts. For example, a retail chain might rely on predictive analytics for forecasting holiday sales and adjusting requirements in such scenarios.
Inventory Optimization
Predictive analysis in supply chain management helps businesses to maintain optimum inventory levels and forecast particular product demands. This is crucial for such companies when deciding whether or not to stock a central warehouse or disperse regional facilities according to localized demand.
Supply Chain Risk Mitigation and Disruption Prediction
Disruption may affect the supply chain at any time through a supplier delay, transport bottlenecks, or unpredicted events. Predictive analytics in supply chain identifies the probability of such disruptions and suggests various mitigation activities so that businesses can continue operating even in volatile market conditions.
Logistics and Transportation Efficiency
Predictive analytics can examine variables such as traffic, weather conditions, and delivery deadlines to optimize shipping routes and schedules, thereby reducing transportation costs, delivering on time, and fulfilling customer satisfaction.
Benefits of Using Predictive Analytics in Supply Chain Management
Cost Reduction through Better Forecasting
Indeed, proper forecasting of demand and sound management of inventory result in minimized operational costs. Deployment of supply chain predictive analytics will reduce both carrying costs for unnecessary inventory, delays in transportation, and stockouts of goods, all financial savings to the enterprise.
Improved Resource Allocation and Efficiency
Predictive Analytics in supply chain management improves resource allocation such as inventory, labor, and transportation in optimal use for enhancing the overall efficiency of a supply chain. Consequently, there are improvements in lead times and deliveries.
Increased Customer Satisfaction and Service Levels
The product availability will truly fulfill their time and location demand by optimizing inventory management through demand forecasting. Hence, improving customer satisfaction, which is paramount to business success in a highly competitive environment where customer loyalty is an important consideration.
Challenges in Implementing Supply Chain Predictive Analytics
Data Quality and Availability Issues
Success in supply chain predictive analytics apparently depends on the quality and the amount of data used. It follows that in case the data are incomplete, old, or incorrect, then the predictions will achieve nothing. The companies have watchdogs that will invest in proper data management and quality assurance processes.
Overcoming Technological and Organizational Barriers
To successfully implement predictive analytics, one needs technical expertise and infrastructure, and organizations with such requirements often learn about the difficulties on the integration of new tools with existing systems. Resistance to Change is also found within the organizations.
Ensuring Privacy and Security of Supply Chain Data
Due to increased reliance on data, it is crucial to ensure protection of data against any unauthorized access or cyber threats. Supply chain data must be ensured security, if customers are to keep their trust and regulations like GDPR are to be adhered to.
Real-World Examples and Case Studies
How Amazon Uses Predictive Analytics for Inventory Management
Amazon uses supply chain predictive analytics to forecast demand for millions of products by analyzing historical data and trends. This helps maintain optimal inventory levels, preventing stockouts and overstocking. The insights also allow Amazon to optimize warehouse storage for better efficiency. Predictive analytics enables faster order fulfillment, reducing delivery times. This approach enhances both operational efficiency and customer satisfaction.
Case Study: Predictive Analytics for Logistics at UPS
UPS uses predictive analytics to optimize delivery routes by analyzing real-time data like traffic and weather. This helps reduce fuel consumption and improve delivery times. Predictive models also allow UPS to anticipate delays and adjust routes proactively. This leads to cost savings and improved efficiency. Ultimately, UPS enhances its service levels while lowering operational costs.
How Retailers Leverage Predictive Analytics for Demand Forecasting
Retailers like Walmart and Target use predictive analytics to forecast sales during peak seasons. By analyzing historical data and trends, they can adjust inventory levels to meet demand. This prevents stockouts while reducing excess inventory. Predictive analytics helps retailers stay competitive by maintaining product availability. It also minimizes the risk of markdowns during peak sales periods.
The Future of Supply Chain Predictive Analytics
Integration with IoT and RFID for Real-Time Tracking
Supply chain predictive analytics looks bright with the advent of incorporating IoT and RFID technologies, enabling the real-time tracking of products, shipments, and equipment. This will make more and more accurate data available for predictions, allowing businesses to respond to issues much faster.
The Impact of AI and Machine Learning Advancements
Advancements in AI and machine learning will allow predictive analytics to assume a more automated and increasingly accurate position. Such advancements will take further steps toward improving decision-making with limited human intervention.
Evolution of Predictive and Prescriptive Analytics in Supply Chains
In fact, prescriptive analytics, which is more advanced than predictive analytics and goes one step ahead to recommend actions, will eventually work together with predictive models in the future. This way, business people will not only predict and know when trends happen, but they will also receive information on what needs to be done to create such a situation that they would want to achieve it.
How to Implement Supply Chain Predictive Analytics in Your Organization
Step-by-Step Guide to Implementing Predictive Analytics
To successfully implement predictive analytics, businesses should:
- Identify business goals and areas for improvement.
- Gather relevant data from across the supply chain.
- Choose the right tools for data integration and analysis.
- Train teams to understand and act on predictive insights.
- Monitor and refine the models to ensure continuous improvement.
Tools and Technologies for Predictive Analytics in Supply Chain
There are various tools available, such as SAP Integrated Business Planning (IBP), Oracle Cloud SCM, and IBM Watson Supply Chain, which offer robust predictive analytics capabilities for supply chains.
Key Considerations for a Successful Implementation
When implementing predictive analytics, organizations must ensure they have high-quality data, the right technology stack, and the necessary skills within their teams to maximize the effectiveness of the tool.
Conclusion: Why Supply Chain Predictive Analytics is Key to Your Future Success
Key Takeaways
- Predictive analytics helps make your supply chain more efficient, cut costs, and keep customers happy.
- To make it work, you need good data, the right tools, and a skilled team.
- The future of supply chains will rely on AI, IoT, and real-time data.
Actionable Steps to Start Your Predictive Analytics Journey
Begin by picking one area of your supply chain that could use improvement, gather the data you need, and start using predictive tools to tackle problems before they happen.
At Entrans, we make it easier for businesses to use AI-driven predictive analytics to streamline operations and make smarter decisions. Reach out to us today and see how we can help you get started on optimizing your supply chain for the future.
Stay ahead with our IT Insights
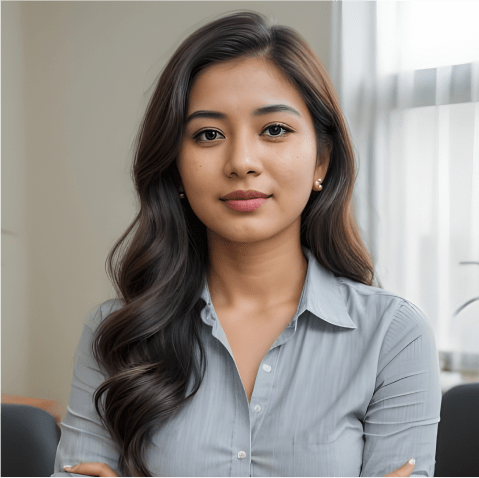
Discover Your AI Agent Now!
An AI Agent Saved a SaaS Company 40 Hours in a Week!