Data Analytics vs. Data Analysis: Key Differences and Use Cases
Data plays a key role in shaping decisions in today's business world. Companies and researchers analyze large amounts of information to uncover useful insights. However, two terms often cause confusion: data analytics and data analysis. While they are closely linked, they serve different purposes in how businesses interpret and act on data-driven insights.
This blog explores data analytics vs. data analysis, breaking down their key differences, real-world applications, and how businesses can make the best use of them. By the end, you’ll know which approach fits your needs and why Entrans is the right choice for data-driven success.
What is Data Analysis?
Data analysis is the process of reviewing, organizing, and making sense of data to find meaningful insights. It involves using numbers and logical methods to spot trends, patterns, and connections in data. Businesses rely on data analysis to learn from past results, measure progress, and make smart choices.
Key Steps in Data Analysis:
- Exploratory Data Review (EDR): Companies examine data to identify patterns and spot unusual trends. In marketing, this helps understand what customers buy by looking at past sales and recognizing seasonal demand shifts.
- Statistical Examination: Businesses study customer details and behavior using statistics. Methods like correlation, variance, and regression help find useful connections in data, leading to better decisions.
- Data Modeling: Predictive models help forecast future trends. Banks, for instance, look at past credit scores to assess loan risks and make better lending decisions.
- Data Visualization: Data is displayed using graphs, charts, and dashboards. A retail store might analyze sales heatmaps to find the busiest shopping hours and adjust staff schedules to match demand.
Example:
A retail business studying sales records might notice that online purchases rise over weekends. This finding helps them adjust their online ads to attract more customers and boost revenue.
What is Data Analytics?
Data analytics is a wide-ranging approach that involves gathering, organizing, and studying data to uncover useful patterns. It includes different methods, such as machine learning, forecasting, and automated processes, to support smarter choices.
Key Steps in Data Analytics:
- Defining the Problem: Companies define their goals before analyzing data. For example, an online store might focus on improving the number of customers who complete their purchases.
- Data Gathering: Data comes from structured sources like databases, unstructured sources such as social media, and semi-structured formats like JSON files.
- Data Organization and Cleaning: The data is arranged in an easy-to-use format, and any errors are fixed to keep it accurate.
- Advanced Analytics: Machine learning techniques and statistical methods help find deeper patterns in data. For example, Netflix analyzes what users watch and suggests shows based on their viewing habits.
Example:
A hospital studies patient records to spot health patterns and predict disease outbreaks, helping doctors plan better treatments in advance.
Data Analytics vs. Data Analysis: Key Differences
Data Analytics vs. Data Analysis: Use Cases
Use Cases for Data Analysis
Retail Performance Monitoring
A global fashion retailer, Zara, continuously analyzes its sales data across different locations to understand customer buying trends. By studying past sales reports and inventory turnover rates, Zara identifies which clothing styles perform best in each region. This helps the company optimize its inventory, reduce overstock, and ensure popular products remain available, leading to higher customer satisfaction and increased sales.
Healthcare Patient Reports
Hospitals like Mayo Clinic use data analysis to track patient readmission rates and identify patterns in post-treatment outcomes. By examining past patient records and treatment efficacy, they can pinpoint the factors contributing to frequent hospital visits. This insight helps healthcare providers improve patient care strategies, reduce readmission rates, and develop personalized treatment plans.
Financial Risk Assessment
JPMorgan Chase employs data analysis to review past loan default rates and identify risk factors in lending. By analyzing historical financial data, including credit scores, income stability, and repayment histories, the bank refines its lending policies to mitigate risks. This enables them to make more informed decisions when approving loans and setting interest rates.
Use Cases for Data Analytics
Fraud Detection in Banking
Financial institutions like Citibank use machine learning-powered fraud detection to identify suspicious transactions in real time. By analyzing patterns in customer spending behavior, their analytics systems flag unusual activities, such as a sudden high-value transaction from a different country. These alerts help banks prevent fraud before it occurs, saving millions of dollars annually.
Customer Churn Prediction
SaaS companies like Spotify use predictive analytics to analyze customer behavior and anticipate churn. By tracking user engagement metrics, such as listening frequency, playlist interactions, and subscription cancellations, Spotify’s AI models predict which users are likely to leave. The company then implements personalized offers, such as discounted plans or exclusive content, to retain at-risk customers.
Smart Inventory Management
E-commerce platforms like Amazon leverage predictive analytics to optimize inventory management. By analyzing past sales, seasonal demand trends, and supply chain data, Amazon ensures warehouses are stocked with the right products at the right time. This minimizes delays, prevents stock shortages, and improves overall customer satisfaction. Their AI-powered inventory system also suggests warehouse placements to minimize shipping times.
Which One Do You Need for Your Business?
Deciding between data analytics and data analysis depends on what you need for your business:
- If you need to look at past trends to learn from them, data analysis is the way to go.
- If your focus is on predicting future trends and making better choices, data analytics is more suitable.
- If your business needs both historical insights and future planning, combining both methods is the best approach.
Example:
A retail store might study past sales to understand buying patterns, while data analytics helps forecast future demand and plan inventory more effectively.
Why Choose Entrans for Your Data Needs?
At Entrans, we create data solutions designed to meet your business needs. Whether you need data analysis to find useful insights or advanced analytics to predict future trends, our knowledge helps you make better decisions.
Why Work with Entrans?
- Experienced in AI & Data Science: Our team uses AI-powered analysis to help businesses find useful information that improves decision-making.
- Personalized Data Methods: We create plans that help businesses make smart choices and grow successfully.
- Advanced Tools & Techniques: We use advanced methods like automation, machine learning, and visuals to make working with data easier.
- Extensive Industry Experience: Top companies rely on us to turn data into smarter business decisions.
Conclusion
Knowing the difference between data analysis and data analytics helps businesses make better choices with data. While data analysis looks at past information to find patterns, data analytics helps predict future trends for smarter planning.
By using both methods, businesses can run more efficiently, improve customer experiences, and make smarter decisions with data. Working with Entrans gives you access to advanced analytics tools that fit your needs.
Ready to unlock the power of data? Contact Entrans today!
Stay ahead with our IT Insights
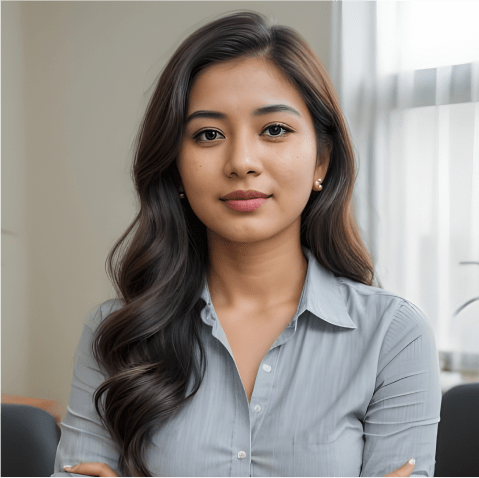
Discover Your AI Agent Now!
An AI Agent Saved a SaaS Company 40 Hours in a Week!