What is IoT Data Analytics? A complete Guide for 2025
IoT data analytics enables businesses to extract valuable insights from the vast amounts of data generated by interconnected devices. By analyzing real-time data, predictive trends, and historical patterns, companies can optimize operations, reduce costs, and make informed decisions. This process involves data collection, processing, analysis, and visualization, helping organizations unlock efficiencies and improve their decision-making. IoT data analytics is increasingly vital for industries like manufacturing, healthcare, and energy, transforming raw data into actionable insights that drive business growth.
What is IoT Data Analytics?
At its core, IoT data analytics deals with converting raw IoT data from interconnected devices into actionable insights. As enterprises incorporate more smart devices, sensors, and machines, the IoT data accumulation becomes an asset. However, it is not just about data collection; the true value lies in its analysis and interpretation to reach smarter decisions.
IoT devices, whether they are a fleet of delivery trucks, a network of smart appliances, or sensors monitoring production lines, need continuous IoT data collection. The IoT data itself, without an analytical engine that can process and interpret the meaning, will practically remain useless. Thus, IoT data analytics steps in to convert real-time analytics in IoT into actionable insights for improving efficiency, reducing costs, and making profits; that's the job it does.
Key Components of IoT Data Analytics
To truly understand how IoT data analytics works, it’s essential to break it down into its core components. Businesses looking to implement IoT analytics should focus on five key areas:
Data Collection
Data collection is the foundational step for the IoT data analysis process. Nowadays, IoT sensors and actuators, like the very latest in environmental measurement techniques, measure anything and everything around the environment: temperature, humidity, location, movement, speed, and many other metrics. These devices then wirelessly transmit the IoT data to selected centralized platforms or cloud storage for further analysis using low-power networks such as Wi-Fi, Bluetooth, or 5G. This stage, as one may term it, lays the groundwork for the accuracy and consistency of IoT data feeding further downstream analytics for IoT.
Data Storage
For storing IoT data, it isn't easy collecting data originating from thousands, if not millions, of devices. While scaling their IoT networks, businesses will need storage solutions that can be high-performance and especially scalable. Most of the time, that includes a combination of on-prem databases with cloud storage or data lakes to have more reliable, secure, and easily accessible storage. A very well data-storing system marries historical IoT data, while the incoming real-time analytics in IoT keeps them safe for future analysis.
Data Processing
Raw IoT data from devices isn’t always clean or formatted correctly for analysis. Thus, cleaning, normalization, and transformation of such data form major processing steps to make it ready for advanced analysis. Data preprocessing includes the identification and removal of outliers, completion of missing records, and transformation of data into its various formats that are compatible with analysis. Without preprocessing, a particular dataset may remain excessively noisy or inconsistent, thereby thwarting trustworthy information extraction from that dataset.
Data Analysis
Here is the essence of IoT data analytics—it provides the actual worth. Data analysis makes use of algorithms and statistical methods to ascertain trends, patterns, and anomalies in IoT data, and companies can leverage historical data analytics in IoT using machine learning and iot predictive analytics to anticipate future behaviors, like when a machine is likely to fail in operation or when an increase in customer demand may occur. The learning from past IoT data by artificial intelligence (AI) may likewise enhance the processes by improving the superb application in prediction and recommendation.
Data Visualization
The last but not the least stage in IoT data analytics is visualization, that is, rendering raw insights into user-friendly formats, i.e., dashboards, charts, and graphs. The visualization tools should be very effective in presenting complex findings in simple and intuitive formats so that the decision-makers can rapidly, if not instantaneously, appreciate the insights and make informed decisions. Real-time dashboards could be used to visualize the live feeds from the IoT data sources, while historical trends could be visualized through a wide range of methods, such as line graphs, pie charts, or even heatmaps, depending on the specifics of the context of the business.
Types of IoT Analytics
IoT analytics is not a one-size-fits-all process. Different types of IoT analytics are applied to solve different business problems. The four primary types of IoT analytics include:
Historical Analytics
Historical analytics refers to analyzing past IoT data to find trends over time. From the assessment of past performance, an organization can have a view of repetitive problems, fluctuations with seasons, or long-term trends. For example, in manufacturing, historical IoT data could tell when machines usually are likely to fail so that organizations will have time to conduct preventative maintenance, avoiding downtime during peak hours. Such analytics for IoT are essential for enhancing business forecasts as well as strategic decision-making.
Real-Time Analytics
In real-time analytics in IoT, data are being analyzed while they are being produced. This is mostly useful in operations where instantly insightful and instantaneous responses are needed. For instance, real-time analytics in IoT manufacturing could monitor the performance of machines and instantly alert operators of abnormal performance or impending failure. Such real-time data feeds will help prevent downtime and optimize the operational performance.
Predictive Analytics
Predictive analytics takes past IoT data as well as real-time information to forecast future events. This kind of analytics in IoT estimates failures in machinery, increases in customer demand, or the arrival of optimum operational conditions. By predicting these outcomes, businesses can then intervene beforehand, such as scheduling maintenance before a machine fails or changing production schedules to accommodate expected demand.
Prescriptive Analytics
While predictive analytics tells businesses what is likely to happen, prescriptive analytics goes ahead to recommend actions to be taken in order to improve outcomes, and then there is prescriptive analytics, which recommends optimal actions to be put in place based on predictive analytics going beyond that process. The techniques generally recommended might suggest fuel-efficient modifications to supply chain routes or alter throughput-optimizing manufacturing schedules, for instance.
IoT Data Analytics Applications in Various Industries
The versatility of IoT data analytics allows businesses across industries to leverage it for optimization and improvement. Here are some key applications:
Manufacturing
IoT Data analytics in manufacturing are used to monitor machine health, calibrate production lines, and even forecast breakdowns. Real-time analytics in IoT ascertain the present performance of machines, while predictive analytics set forth potential breakdowns, thereby allowing companies to go for predictive maintenance and lower any unplanned downtime. Optimizing machine performance is also achieved through IoT analytics at companies such as General Electric and Siemens.
Energy Management
With the use of IoT analytics, energy companies manage energy consumption in real time. Energy companies integrate smart meters and IoT sensors into the energy grid to analyze data analytics for IoT that can minimize electricity waste by improving the management of the grid and maximizing the efficiency of energy distribution. This translates to lower costs and support for sustainability goals through lesser energy consumption and emissions.
Smart Cities
IoT data analytics is essential for traffic management, waste management, and resource optimization in smart cities. For example, Barcelona installed sensors to inform real-time traffic pattern data collection, which will effectively reduce congestion, pollution, and improve the quality of life in the urban environment. Air quality, street lighting optimization, and public transport optimization are also IoT data analytics applications.
Healthcare
IoT data analytics allows healthcare providers to monitor patient health remotely and estimate and predict disease conditions before they get severe. Various wearable gadgets are capable of tracking vital signs like heart rate, blood pressure, and activity level and sending all these real-time data to the health providers. From there, detection and recognition of patterns in predictive analytics are used to identify possible health risks before they require hospital attendance.
Challenges in IoT Data Analytics
While the benefits of IoT data analytics are clear, businesses face several challenges in implementing it successfully:
Data Security and Privacy
IoT devices churn out a huge amount of sensitive IoT data, putting security into serious jeopardy. Data encryption and accessibility to only the authorized users should be used to protect the privacy as well as provide compliance with regulations like GDPR.
Data Quality
IoT data can be very noisy or even fragmented, increasing the necessity of data cleaning and quality checks. Poor-quality IoT data triggers unreliable analytics for IoT and poor decision-making. Proper measures should be taken by businesses to ensure that data analytics for IoT is accurate, consistent, and reliable to facilitate these measures.
Scalability
As the Internet of Things networks grow, the magnitude of generated IoT data increases tremendously. A considerable investment in scalable infrastructure for businesses is completely unavoidable in the IoT data collection process without degrading performance. This may mean cloud storage platforms, edge computing, or hybrid solutions for alternative IoT data management.
Interoperability
IoT gadgets are made by various companies and often have several protocols. For the potential of IoT data to be fully realized, it is crucial to make those devices communicate and link them together in an integrated analytics for IoT framework.
Best Practices for Implementing IoT Data Analytics
To successfully implement IoT data analytics, businesses should follow these best practices:
- Start with a Clear Data Strategy
Define the business goals, data collection methods, and analysis techniques to ensure that the analytics for IoT efforts align with your organization’s objectives. - Ensure Data Security and Privacy
Implement strong encryption and access control measures to safeguard sensitive IoT data and comply with regulations like GDPR. - Invest in Scalable Infrastructure
As your IoT network grows, ensure that your analytics infrastructure can handle the increased data volume without compromising performance. - Continuously Monitor and Optimize
IoT data analytics is not a one-time effort. Regularly monitor the IoT data, adjust analytics models, and optimize systems to ensure continued success.
Future Trends in IoT Data Analytics
The future of IoT data analytics is bright, with several emerging trends set to shape the landscape in the coming years. These trends will not only drive greater efficiency but also unlock new opportunities for businesses that adopt them early.
AI and Machine Learning Integration
The advent of artificial intelligence and machine learning will add power to the automation of IoT data analysis. When companies rely on AI-empowered models, they can discern deeper insights, identify hard-to-perceive patterns, and provide faster, more accurate predictions.
For example, predictive maintenance can be improved by AI analytics based on historical IoT data, continuously optimizing its predictions on the likelihood of equipment failure. This will optimize unplanned downtime and operational costs.
Edge Computing
The IoT data generated from IoT devices will continue growing, which will inevitably make edge computing popular. It reduces the amount of raw IoT data sent to a central server for processing, thus reducing latency and bandwidth, since many processes would be done closer to the source of the data.
Edge computing is beneficial for applications that require real-time analytics in IoT in an immediate decision-making process based on data from sensors and cameras, such as autonomous vehicles and smart manufacturing.
Predictive and Prescriptive Analytics
Predictive analytics and prescriptive analytics will bring about a revolution in IoT data analytics. Predictive analytics foresee future trends based upon historical IoT data and can therefore become even more accurate; prescriptive analytics will therefore provide businesses with actionable recommendations depending on the predicted outcome.
Like in this example, prescriptive analytics would suggest the best action in terms of managing energy consumption for smart grids so as to increase energy efficiency and decrease costs.
Why Choose Entrans for IoT Data Analytics?
At Entrans, we understand the potential of IoT data analytics and the challenges businesses face when implementing it. With our expertise in data collection, processing, and analysis, we help organizations transform their IoT data into actionable insights that drive decision-making and operational efficiency.
What Makes Entrans Stand Out?
- Tailored Solutions: We customize IoT data analytics strategies to meet the unique needs of each industry, ensuring that businesses get the most value from their data.
- Proven Expertise: Our experience spans across industries like manufacturing, healthcare, and energy, where we’ve successfully implemented IoT analytics to drive growth.
- Scalable Infrastructure: We design analytics solutions that scale with your business, ensuring long-term success as your IoT network expands.
- End-to-End Support: From data collection to real-time insights, Entrans offers comprehensive IoT analytics services that ensure every aspect of your system is optimized for success.
Ready to transform your IoT data into actionable insights? Schedule a free 30-minute consultation today!
Stay ahead with our IT Insights
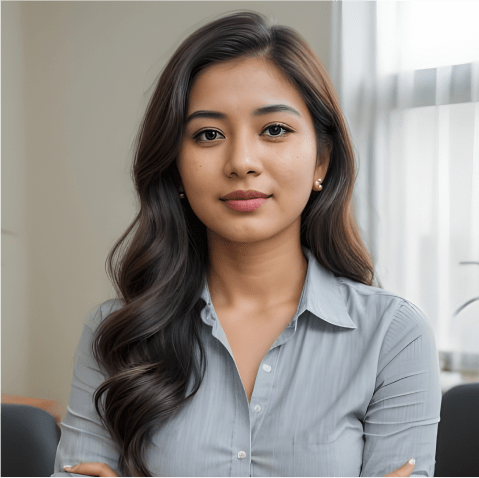
Discover Your AI Agent Now!
An AI Agent Saved a SaaS Company 40 Hours in a Week!