Unlock the Power of Retail Analytics: Key Tools and Strategies for 2025
Retail analytics empowers businesses to make data-driven decisions that optimize inventory, enhance sales forecasting, and personalize customer experiences. By analyzing sales data, customer behavior, and supply chain movements, retailers can anticipate trends, reduce waste, and improve profitability. Retail predictive analytics and prescriptive insights are becoming essential tools for staying competitive, helping businesses forecast demand and adjust strategies in real-time. In 2025, as omnichannel retailing grows, leveraging data analytics for retail will be crucial for maintaining an edge in a rapidly evolving market. Retail analytics isn't just a tool—it's the key to informed, effective decision-making in today's retail environment.
What is Retail Analytics?
Retail analytics is the practice of using data to drive decisions across the retail value chain. It involves analyzing information from multiple sources—sales transactions, customer interactions, supply chain movements, online behaviors, and even weather trends—to understand what’s happening in the business and how to improve it.
It goes beyond simple reporting. True retail data analytics includes predictive capabilities (what will happen), diagnostic insights (why something happened), and prescriptive recommendations (what should be done next). It connects data from both digital and physical channels, offering retailers a comprehensive view of operations and customer behavior.
According to a Deloitte study, data-driven retailers are 23 times more likely to acquire customers, six times as likely to retain them, and 19 times more likely to be profitable than their less-analytic peers. Retail business analytics isn't a luxury anymore—it's a business necessity.
Why is Retail Analytics Important in 2025?
The retail industry in 2025 is defined by uncertainty. Consumer behavior is more fragmented than ever. One customer might browse on mobile, compare on desktop, and purchase in-store. Another may never step foot in a physical store again. At the same time, global supply chains remain volatile, and inflation pressures force every pricing decision to be surgical.
Retail analytics offers a lifeline. It helps businesses respond to trends in real-time and anticipate changes before they happen. Take demand forecasting, for instance. With retail predictive analytics, companies can now analyze years of sales data, weather patterns, holidays, and even social media chatter to accurately predict future demand. This prevents overstocking, reduces markdowns, and keeps shelves full of the right products.
McKinsey reports that retailers leveraging analytics in supply chain planning saw a 5-10% increase in gross margin. In a low-margin industry, that’s game-changing.
Types of Retail Analytics
Retail analytics can be grouped into four main types, each with its own purpose and value. These types build on each other, evolving from understanding past performance to recommending future actions.
Descriptive Analytics
Descriptive analytics focuses on summarizing past data to provide insights into what happened within a retail business. It includes analyzing historical data like sales reports, inventory levels, customer visits, and transaction details. Retailers use business intelligence (BI) dashboards to visualize this data, making it easy to track key performance indicators (KPIs). This helps businesses understand trends such as best-selling products or peak shopping times. Retail analysis is essential for evaluating past performance and guiding operational decisions, like restocking or adjusting marketing campaigns.
Diagnostic Analytics
Diagnostic analytics helps retailers understand the reasons behind certain outcomes. For example, if a retail business sees a drop in sales, diagnostic analytics will look deeper into factors such as changes in foot traffic, pricing strategies, or supply chain disruptions. It uses statistical techniques, correlation analysis, and retail data analysis to identify underlying causes. By understanding why something happened, retailers can fix issues, like adjusting pricing or improving product displays. This type of analysis is vital for addressing performance gaps and ensuring the business is operating efficiently.
Predictive Analytics
Predictive analytics uses historical data and statistical models to forecast future trends or events. Retailers apply this type of analytics to predict customer demand, sales performance, and inventory needs. For instance, predictive models can forecast demand for a product based on previous sales patterns, seasonal trends, and external factors like weather. Retailers can also use predictive analytics to identify potential customer churn and take proactive measures to retain them. By anticipating what might happen, businesses can make more informed decisions about inventory management, promotions, and staffing.
Prescriptive Analytics
Prescriptive analytics goes beyond prediction by providing actionable recommendations on what actions to take. It combines insights from predictive analytics with AI and simulation techniques to optimize strategies for pricing, promotions, and inventory allocation. For example, it can recommend the best price for a product based on factors such as demand elasticity, competition, and customer behavior. Retailers use prescriptive analytics to fine-tune their operations, improve customer experiences, and maximize profitability. This type of analytics helps businesses turn insights into tangible actions that drive performance and growth.
Real-World Applications of Retail Analytics
Let’s talk about where retail analytics delivers value in the real world.
Optimizing Fresh Produce Ordering with Predictive Analytics
A grocery chain in the U.S. used customer data, weather forecasts, and regional buying patterns to optimize its fresh produce ordering. As a result, waste dropped by 30%, and customer satisfaction improved due to better stock availability.
Tailoring Inventory to Local Demand in Fashion Retail
In fashion retail, analytics in retail helps brands decide what styles and sizes to stock at each location. Using local sales history and demographic trends, a major sportswear brand reduced stockouts by 20% while also cutting excess inventory.
Personalizing the E-Commerce Experience with Web Analytics
E-commerce players use retail web analytics to personalize the customer experience. Platforms analyze click paths, time on page, abandoned carts, and purchase history to tailor product recommendations, boosting conversion rates by up to 15%.
Enhancing Store Operations with Video Analytics and Heatmaps
Analytics in retail industry also supports fraud detection, optimized staffing schedules, targeted promotions, and even in-store layout planning using video analytics and heatmaps.
Tools and Technologies Used in Retail Analytics
Retailers today rely on various tools and technologies to gather, analyze, and act on data. These tools help businesses make data-driven decisions, optimize operations, and improve customer experiences.
Business Intelligence (BI) Tools
BI tools like Tableau, Power BI, and Qlik help retailers visualize trends and track KPIs across operations. These tools allow for real-time analysis and reporting of sales, inventory, and retail customer analytics. Retailers can create dashboards and reports to uncover valuable insights from data. By integrating various data sources, BI tools enable better decision-making. They are essential for tracking performance and improving business outcomes.
Customer Data Platforms (CDPs)
Salesforce, Segment, and Bloomreach centralize customer data from multiple touchpoints, creating unified customer profiles. CDPs help retailers deliver personalized experiences by analyzing behavioral data across channels. These platforms enhance customer segmentation and targeted marketing efforts. By unifying data, retailers can improve customer engagement and loyalty. CDPs are crucial for driving personalized marketing strategies.
Predictive Analytics Engines
SAS, Google Cloud Retail, and Azure AI help retailers forecast demand, predict customer behavior, and optimize inventory. These predictive tools analyze historical data to anticipate future trends. Retailers can use this data to plan inventory, promotions, and staffing. Retail predictive analytics helps businesses respond proactively to changes in demand. These tools improve decision-making by providing accurate forecasts.
Retail-specific Analytics Solutions
Retail-specific solutions like Oracle Retail, Retalon, and Blue Yonder are tailored to the needs of retailers. These platforms integrate with POS, supply chain, and merchandising systems. They provide insights into inventory management, pricing, and customer demand. Retail-specific solutions help optimize operations from the warehouse to the storefront. These tools support businesses in maximizing profitability and efficiency.
Cloud Data Warehouses
Snowflake and BigQuery offer cloud-based data storage solutions that consolidate large volumes of data. These platforms enable seamless integration of sales, retail customer data, and inventory data. Retailers use cloud data warehouses to generate insights from diverse data sources. They provide scalability and speed for data processing and analysis. Cloud warehouses support advanced analytics and machine learning capabilities for deeper insights.
When to Upgrade Your Retail Analytics Stack
If your team is still juggling spreadsheets, it’s time to ask some hard questions:
- Are we constantly reacting to stockouts or overstocks?
- Do different departments use inconsistent data?
- Are decisions driven more by intuition than data?
- Are we missing opportunities for personalization or targeted promotions?
According to Gartner, companies that modernize their analytics stack see up to 30% faster decision-making and a 20% improvement in operational efficiency. Delaying the upgrade means falling behind.
Future of Retail Analytics
As AI becomes embedded into everyday tools, analytics will shift from dashboards to decisions. Store associates will receive real-time prompts on what to restock, when to engage a shopper, or how to resolve a complaint.
Retail predictive analytics will also become more contextual. Instead of saying, “Your store may run out of Product X,” systems will suggest, “Increase inventory for Product X by 20% in Location A next week due to forecasted temperature rise and local event traffic.”
Analytics will move beyond insights and become invisible—powering every recommendation, every campaign, every store layout decision.
How Entrans Empowers Retailers with Advanced Analytics
At Entrans, we help retailers transform fragmented data into actionable intelligence. Our analytics consulting and technology services are tailored for modern retail environments, whether you're running 10 stores or 10,000 SKUs online.
We unify your sales, inventory, customer, and supply chain data to uncover patterns you can act on—from reducing returns and forecasting demand to optimizing promotions across geographies. Our strength lies in helping you adopt predictive and prescriptive analytics without needing a PhD in data science.
Whether you’re just starting your analytics journey or scaling your current stack, Entrans equips you with the clarity, tools, and support to make data your biggest advantage.
Want to make data-driven decisions that fuel growth? Book a free 30-minute consultation call today!
Stay ahead with our IT Insights
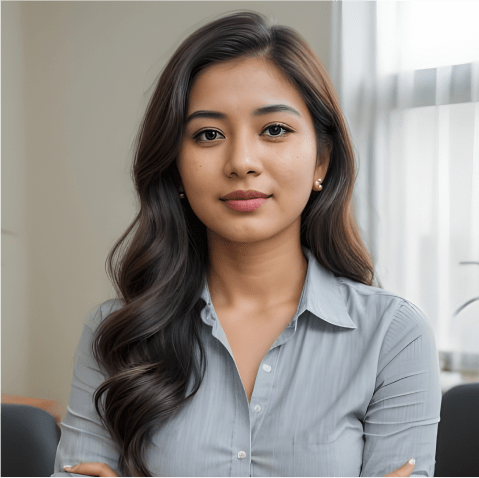
Discover Your AI Agent Now!
An AI Agent Saved a SaaS Company 40 Hours in a Week!