Generative AI Meets IoT: Transforming Connected Devices with Smarter Solutions
The secret behind agility across processes? IoT processes powered by generative AI. Although overlooked it can help understand how your business moves with information.
IoT is used in Retail, Healthcare, and a wide range of other industries. And the fact is that helps you turn data into insightful info a lot quicker.
Generative AI improves this and we’ll show you how…
What Does AI in IoT Mean?
Generative AI in IoT improves it by making real-time data insights smarter and automating complex decisions. Alongside this, Predictive analytics reduces downtime, while adaptive learning improves efficiency. The result results in higher ROI and a stronger competitive edge.
One example of this could be using generative AI to forecast demand shifts in energy usage. This means less blackouts and lower usage of fossil fuels! And this is just one example of how it can change one industry.
How Does Generative AI in IoT Work?
Generative AI in IoT works by combining the power of generative models, which can create new data or content, with the connectivity and data-gathering capabilities of IoT devices.
This combination allows a range of new possibilities, including:
- Step 1 - Data Acquisition and Preprocessing: IoT devices collect various data from the physical world, such as sensor readings, images, and audio. This data is then preprocessed to clean, normalize, and formatted.
Step 2 - Generative Model Training: A generative AI model, such as a Generative Adversarial Network (GAN) or a Variational Autoencoder (VAE), is trained on a large dataset of relevant IoT data. The model understands patterns in the data to generate new data that resembles the original. - Step 3 - Generative AI Inference and Application: The trained generative AI model can then be used to generate new data instances, such as synthetic sensor readings or images, or to augment existing data. This generated data can be used for various purposes as well based on need.
- Step 4 - Data Acquisition and Preprocessing: The generative AI model can be used alongside existing IoT systems to provide new functionalities, such as anomaly detection, predictive maintenance, or data anonymization. This can done through cloud computing, edge computing, or on-device processing.
- Step 5 - Continuous Learning and Improvement: The generative AI model can continuously learn and improve as new data is collected from IoT devices. This helps the model to adapt to changing conditions with more accurate and relevant results over time.
What are the Main Challenges in Traditional IoT?
1. Difficulty in Scalability for Growing Companies
Traditional IoT systems often struggle to handle the increasing volume of data and devices as companies grow. This can lead to performance bottlenecks, increased latency, and difficulty in managing and analyzing data effectively. For example, a smart home system with a few devices may work fine, but as you add more sensors, cameras, and appliances, the system may become slow and unreliable.
2. Difficulty Making Decisions Immediately
Traditional IoT systems often rely on centralized cloud processing, which can introduce delays in decision-making.
This latency can be problematic for applications that require real-time responses, such as autonomous vehicles or industrial automation. For instance, a self-driving car needs to make split-second decisions based on sensor data, and any delay could lead to accidents.
3. Lack of Data Makes Training Models Challenging
Building effective AI models for IoT applications often requires large amounts of diverse data. However, in many cases, real-world data can be scarce, expensive to collect or contain sensitive information.
This can hinder the development and deployment of AI solutions for IoT. One illustration for this would be training a model to detect anomalies in medical devices that may require access to patient data, which raises privacy concerns.
4. IoT Data is Sensitive and Needs to Be Encrypted First
IoT devices often collect and transmit sensitive data, such as personal information, financial transactions, or industrial control signals. This data needs to be protected from unauthorized access and cyberattacks.
Traditional IoT systems may lack robust security measures, making them vulnerable to data breaches and other threats. For example, a smart city network that manages traffic lights and surveillance cameras could be targeted by hackers to disrupt critical infrastructure.
Using a Hybrid Generative AIoT Framework
Predictive Maintenance
Generative AI models can be used to predict when equipment is likely to fail, allowing for proactive maintenance and reducing downtime.
These models can analyze sensor data, historical maintenance records, and other information to identify patterns and anomalies that indicate potential failures.
This could look like a factory using AI to predict when a machine is likely to break down and schedule maintenance before it disrupts production.
Anomaly Detection
Generative AI models can be trained to detect unusual patterns or events in IoT data that may indicate anomalies or security threats.
These models can learn the normal behavior of a system and identify deviations that could signal problems. Smart grids in energy and tech industries can use AI to detect power fluctuations that could indicate equipment malfunction or cyberattacks.
Synthetic Data Generation
Generative AI models can create synthetic data that mimics real-world data but does not contain sensitive information.
This synthetic data can be used to train AI models for IoT applications without compromising privacy or security.
This could be something like a healthcare provider using AI to generate synthetic patient data to train a model for disease prediction without revealing actual patient records.
Benefits of Generative AI in IoT
1. Better Prediction Accuracy
Generative AI models can often achieve higher accuracy in predicting future events or states in IoT systems compared to traditional methods. This is because they can learn complex patterns and relationships in data that may not be apparent to humans or simpler algorithms.
For example, a generative AI model could more accurately predict equipment failures in a factory by considering a wider range of factors, such as sensor readings, maintenance history, and environmental conditions.
2. Cheaper Maintenance Costs
By predicting failures and enabling proactive maintenance, generative AI can help reduce maintenance costs in IoT systems. This is because it can help avoid costly downtime and repairs by identifying potential problems before they occur.
For example, a smart building could use AI to predict when HVAC systems need maintenance, preventing breakdowns and extending the lifespan of the equipment.
3. Fewer False Positives
When Detecting Anomalies Generative AI models in IoT can be trained to be more sensitive to true anomalies while reducing false positives. This is because they can learn the normal behavior of a system more accurately and identify deviations that are truly significant.
For example, a security system using AI could be better at distinguishing between actual threats and harmless events, reducing unnecessary alerts, and improving response times.4. Lower Latency (Quicker Decisions)Generative AI models when used in IoT can be deployed on edge devices, enabling faster decision-making and reducing reliance on cloud communication.
This can be crucial for applications that require real-time responses, such as autonomous vehicles or industrial automation. For example, a self-driving car with AI on board could react more quickly to obstacles or changing road conditions.
5. A Lot More Energy Efficiency
Generative AI in Iot can be used to optimize energy consumption in IoT systems by predicting usage patterns and adjusting settings accordingly.
This can lead to significant cost savings and environmental benefits. For example, a smart home could use AI to learn the occupants' habits and adjust lighting, heating, and cooling to minimize energy waste.
6. Data Generation and Augmentation Takes a Lot Less Time
Generative AI can quickly generate large amounts of synthetic data for training other AI models or augmenting existing datasets.
This can save time and resources compared to collecting and labeling real-world data. For example, a company developing a new sensor could use AI to generate synthetic data to test its performance under various conditions without having to deploy physical sensors in the field.
What are Some Potential Roadblocks to Using Gen AI in IoT
- Data Scarcity and Quality: Generative AI models require large amounts of high-quality data for training. In some IoT domains, sufficient data may be scarce or expensive to collect, hindering model development. Additionally, real-world IoT data can be noisy or incomplete, affecting model accuracy.
- Computational Resources: Training and deploying generative AI models can be computationally intensive, requiring significant processing power and memory. This can be a challenge for resource-constrained IoT devices or edge deployments. Optimizing models for efficiency is crucial.
- Security and Privacy: Generative AI models can be vulnerable to attacks or manipulation, potentially compromising IoT systems or generating misleading results. Protecting models and data from unauthorized access or tampering is essential.
- Explainability and Interpretability: Understanding how generative AI models make decisions can be challenging, especially for complex models. This lack of transparency can hinder trust and adoption, particularly in safety-critical IoT applications.
- Ethical Considerations: Generative AI models can raise ethical concerns, such as the potential for bias, misuse, or unintended consequences. Careful consideration of these issues is needed to ensure responsible development and deployment.
Partnering With Entrans for Custom Generative AI
Working with experts allows you to optimize AI models for better IoT performance while proactively identifying and mitigating security risks, and safeguarding sensitive data.
This can help you with data compliance laws, smoother data pipelines that are validated properly, and also with removing biases in generative AI applications.
Trying to speed up your processes with Generative AI? Don’t hesitate to reach out for help!
Stay ahead with our IT Insights
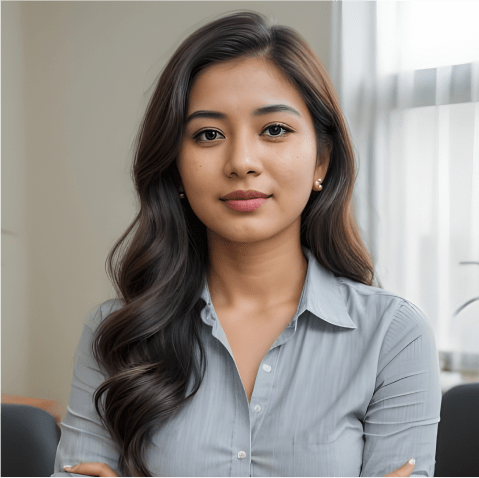
Discover Your AI Agent Now!
An AI Agent Saved a SaaS Company 40 Hours in a Week!