A Complete Guide for Generative AI Enterprise Architecture
Using generative AI in enterprise architecture can seem overly complicated. But the fact is that although it may seem challenging to implement, once done, it creates a system that continually improves itself.
Wondering how you can use generative AI to boost productivity and compliance in your company? Well, we’ll cover everything you need to know about automation.
How Is Generative AI Incorporated Into Enterprise Architecture?
Generative in enterprise architecture is used to automate processes, validate data, and use internal data to optimize prediction references and issues.
This can be brought into any process based on requirements and usage. In enterprise architecture, generative AI offers unparalleled automation. This means that consumer insight, analytics, and processes can be validated and monitored in real-time.
In the large scheme of things, this reduces the need for manual intervention spent on creating reports and ensuring compliance. Which for management and businesses means more time focusing on your service and customer experience.
How Generative AI Can Improve Enterprise Architecture
Improved Integration between Workflows and ERP
Generative AI enhances workflow integration by streamlining connections between systems like ERP, ensuring seamless data flow and real-time updates across processes.
This technology enables ERP systems to automatically adjust to changes in workflows, reducing bottlenecks and improving operational efficiency. Generative AI ensures that workflows are continuously optimized, minimizing manual oversight and enhancing accuracy in data exchanges.
In the broader perspective, this reduces downtime and improves overall productivity, allowing management to focus on strategic decisions. By improving workflow and ERP integration, businesses can deliver better services and maintain strong operational performance.
Better Compliance, Risk Management, and Fraud Detection
For compliance, risk management, and fraud detection automating data validation and monitoring processes in real-time is a process that can be streamlined with generative AI.
It can be seamlessly integrated into existing workflows to continuously assess risks and ensure regulatory compliance. Generative AI helps identify anomalies and potential fraud patterns before they escalate, reducing the need for manual review.
In the broader context, this minimizes legal and financial risks while freeing up resources. For businesses, this translates to more time dedicated to growth and customer experience, with the added assurance of secure, compliant operations.
Predict Consumer Preferences Based on Historical Data
Generative AI in enterprise architecture can predict consumer preferences by analyzing historical data, enabling businesses to anticipate trends and customer needs.
This capability can be embedded into various processes to offer personalized insights, improving decision-making in real-time. With generative AI, enterprises can refine their strategies by validating consumer behavior and patterns through continuous data analysis.
In the larger context, this reduces the need for manual research, allowing businesses to respond quickly to market changes. For management, this means more time focusing on enhancing customer satisfaction and refining service offerings based on precise consumer insights.
Enhanced Maintenance and Logistic Management
Generative AI in enterprise architecture enhances maintenance and logistics management by automating data analysis and predicting equipment needs or potential disruptions.
This technology can be applied to various processes to ensure timely maintenance and optimize logistics operations. In enterprise architecture, generative AI offers real-time monitoring of assets and supply chains, improving decision-making and resource allocation.
In the broader context, this reduces manual oversight and minimizes delays, resulting in more efficient operations. For management, this means increased uptime and smoother logistics, allowing focus on strategic improvements and better service delivery.
Upgraded CRM Frameworks and Operations
Generative AI in enterprise architecture enhances CRM frameworks by automating customer data analysis and optimizing engagement strategies.
This can be integrated into any CRM system to provide real-time insights into customer behavior, preferences, and interactions. With generative AI, businesses can personalize communications and streamline customer support, ensuring a seamless experience for clients.
In the larger scheme, this reduces the need for manual data management and report generation, allowing companies to focus more on building stronger customer relationships. For management, this means more time to refine operations and improve overall customer satisfaction.
Better Data Preparation and Validation Frameworks
Generative AI in enterprise architecture improves data preparation and validation frameworks by automating data cleansing and ensuring accuracy in real time.
This technology can be applied to any data-driven process, making sure that information is consistently validated and ready for analysis. With generative AI, enterprises can streamline data workflows, reducing errors and manual interventions in the preparation stages.
In the larger context, this minimizes time spent on manual data validation, allowing businesses to focus on deeper insights and decision-making. For management, it means more efficient operations and enhanced confidence in the accuracy of business data.
Challenges of Implementing Generative AI in Enterprise Architecture
Complexity in Integrating Generative AI
Integrating generative AI into enterprise architecture can be complex due to the need for advanced infrastructure and compatibility with existing systems.
This complexity arises from aligning AI models with diverse workflows, ensuring data integrity, and managing real-time processes. In enterprise environments, generative AI requires seamless integration across multiple platforms to deliver its full potential.
In the larger context, this increases the demand for specialized skills and robust IT frameworks. For management, this means balancing the benefits of AI-driven automation with the challenges of technical adaptation, ensuring smooth operations and minimizing disruption to business functions.
Additional Security Requirements - Encryption and Access Control
Implementing generative AI in enterprise architecture introduces new security challenges, particularly around encryption and access control. Advanced encryption is crucial to safeguard sensitive data from unauthorized access and breaches.
Access control must be tightly regulated, ensuring that only authorized users interact with AI-driven systems. Inadequate controls can expose vulnerabilities, leading to data leaks or exploitation of AI-generated insights.
This requires continuous monitoring and updating of security protocols, aligned with evolving threats. Ultimately, these measures help protect critical business assets, allowing organizations to confidently harness AI without compromising security.
Large Quantities of High Quality and Validated Data Required
Generative AI in enterprise architecture requires large volumes of high-quality, validated data to function effectively. The AI models depend on accurate, diverse datasets to generate reliable insights and predictions.
This data must be clean, relevant, and continuously updated to avoid skewed results or flawed outcomes. In enterprise settings, ensuring data quality can be resource-intensive but is crucial for AI's success.
The absence of validated data increases the risks of errors and inefficiencies, leading to misinformed decision-making. By prioritizing data integrity, businesses can fully leverage generative AI's potential to optimize processes and drive innovation.
Complex Governance Frameworks for Compliance with Industry Regulations
Implementing generative AI in enterprise architecture demands complex governance frameworks to ensure compliance with industry regulations. These frameworks are essential for managing the ethical use of AI and protecting sensitive data.
Incorporating AI requires businesses to navigate various legal and regulatory standards, which can be challenging without structured governance in place. Failure to meet these requirements could result in significant legal risks or penalties.
Proper governance not only ensures compliance but also helps maintain the integrity of AI processes. This allows businesses to leverage AI while adhering to regulatory guidelines and mitigating risks.
Stay ahead with our IT Insights
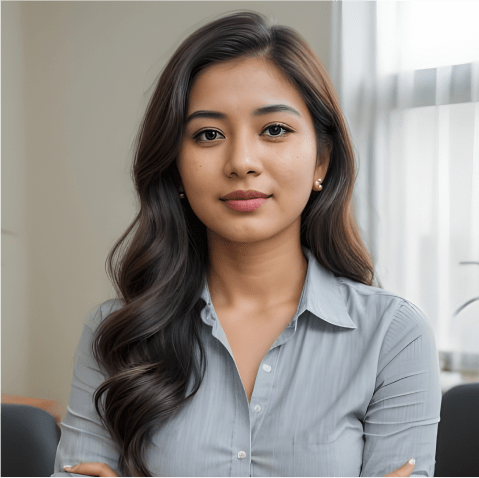
Discover Your AI Agent Now!
An AI Agent Saved a SaaS Company 40 Hours in a Week!