What is Data Analytics? A Complete Guide for 2025
It doesn't matter if you're a traditional or digital enterprise - odds are, there’s a wealth of information and data in your company that can help you improve.
This can be for better supply chain management, identity fraud detection, or even to understand your customers and market better!
Data analytics presents businesses with the chance to make better decisions. So, to help, in this article, we’ll cover what is data analytics and how it can help you.
What is Data Analytics?
Data Analytics represents the multifaceted activity of examining unprocessed information to gain understanding. This involves applying algorithmic or mechanical procedures to extract insights.
Data Analytics methods assist us in revealing patterns and metrics that might otherwise remain hidden. This deals with a broad spectrum of techniques and tools, from simple spreadsheets to intricate statistical models and algorithms.
Why is Data Analytics Important?
Data Analytics has become indispensable for companies across all sectors due to its power to bring about fundamental change. Here are some key reasons why Data Analytics holds such significance:
- Improved Decision-Making: Data Analytics aids those in leadership to perceive current situations, utilizing actual figures and facts. Moreover, by employing past information, you can identify patterns and make more informed choices. This assists in figuring out which products to develop, how to communicate their value, and how to manage operations effectively.
- Boosted Productivity: Data Analytics allows you to inspect processes and identify problems swiftly. With this, you can resolve those issues, accomplish more with fewer resources, and refine workflows. By doing this, you lower expenses, increase output, and observe a rise in performance.
- Deeper Customer Understanding: Knowing customer preferences and dislikes is foundational. Data Analytics allows you to become acquainted with your clientele. With this understanding, you can adjust your marketing to suit them. Data Analytics also supports improvements to your service and the creation of products that people genuinely desire.
- Discovery of New Opportunities: Through Data Analytics, you can identify emerging markets, foresee upcoming trends, and locate avenues for expansion.
- Risk Management: Data Analytics assists you in spotting problems before they occur, assessing their potential impact, and addressing them. Also, predictive deciphering can forecast future dangers, allowing businesses to take proactive steps to lessen potential losses.
- Competitive Advantage: If you use your data effectively, you can perceive aspects your rivals do not, make superior decisions more rapidly, and simply outperform them. Companies that appropriately use their data assets can gain insights that their competitors frequently overlook.
What are the Categories of Data Analytics?
Data Analytics can be divided into four main categories, namely descriptive Analytics, diagnostic Analytics, predictive Analytics, and prescriptive Analytics.
- Descriptive Analytics: What is the function of descriptive Analytics? It aims to understand past occurrences. It involves finding the reasons behind trends and patterns. You might employ data mining, examine relationships, and identify the primary cause of an event. This aids in understanding historical trends, spotting patterns, and tracking key performance indicators (KPIs). Examples include sales reports, website traffic summaries, and financial statements.
- Diagnostic Analytics: Diagnostic Analytics focuses on uncovering the reasons behind trends and patterns. You might use data mining, explore correlations, and pinpoint the fundamental reason for something. This includes correlation analysis and root cause analysis. For example, you might use it to understand why sales declined in a specific region or why more customers discontinued their service.
- Predictive Analytics: Predictive Analytics employs historical data, mathematical formulas, and machine learning to anticipate future events. Predictive Analytics finds application in various areas, including sales forecasting, risk assessment, fraud detection, and customer attrition prediction.
- Prescriptive Analytics: Prescriptive Analytics stands as the most advanced form of Data Analytics. This combines probability assessment with methods to improve outcomes, testing different possibilities to see what yields the best results. Prescriptive Analytics addresses the question, "What course of action should we take?" Examples include setting optimal prices, managing inventory levels, and executing marketing campaigns.
These four categories of Data Analytics build upon one another, with each supplying information for more valuable choices.
Key Advantages of Data Analytics
Data Analytics presents a wide array of advantages to entities that put it into action effectively. Here are some of the most noteworthy benefits:
- Fact-Based Decision-Making: Arguably, the primary advantage of data examination is its capacity to support decision-making with factual information. By supplying objective viewpoints derived from Data Analytics, it lessens reliance on intuition.
- Improved Operational Effectiveness: Data Analytics assists companies in identifying operational and procedural shortcomings. Examining data can reveal bottlenecks, repetitive tasks, and areas where resources are not being used optimally.
- Growth in Revenue: By understanding customer behaviors and market trends, Data Analytics can assist businesses in refining their sales and promotional activities. This can lead to increased sales, better customer retention, and greater profitability.
- Better Customer Interactions: Data Analytics assists businesses in personalizing their interactions with customers. By examining customer information, companies can adjust products, services, and promotional messages to individual preferences, resulting in greater customer satisfaction and loyalty.
- Competitive Edge: Entities that effectively use Data Analytics gain a competitive advantage. They can react more swiftly to market shifts, identify new opportunities, and make more informed strategic choices than their competitors.
- Risk Reduction: Data Analytics assists entities in identifying and assessing potential risks. By analyzing past information and market trends, businesses can anticipate problems and take proactive steps to control them.
- Innovation: Data Analytics can reveal hidden patterns and insights that spark innovation. By analyzing customer feedback, market research, and other data sources, companies can identify unmet needs and develop new products and services.
Data Analytics Instruments and Technology
Working in Data Analytics necessitates familiarity with a diverse set of instruments and technologies, each suited to different types of tasks. These can range from simple uses to advanced enterprise platforms.
- Spreadsheets: Instruments like Microsoft Excel and Google Sheets continue to play a central role in routine analysis. They are frequently used to sort, filter, and calculate data rapidly when dealing with smaller datasets.
- SQL (Structured Query Language): SQL remains a standard for querying and managing relational databases. It allows analysts to extract specific information, combine different datasets, and perform complex operations on the data.
- Programming Languages: Python and R are commonly chosen for statistical work, machine learning, and creating visuals. They both come with extensive collections of libraries designed for analytical tasks.
- Data Visualization Instruments: Instruments like Tableau, Power BI, and QlikView simplify the creation of interactive dashboards and the visual sharing of insights. These assist decision-makers in understanding data trends without needing to interpret code or raw numbers.
- Big Data Platforms: Technologies like Hadoop and Spark are designed to handle data at scale. By operating across distributed systems, they allow teams to address datasets that would otherwise be too large for efficient processing.
- Cloud-Based Analytics: Platforms from AWS, Google Cloud, and Azure grant businesses access to powerful services for storing, analyzing, and modeling data—all without relying on local setups. These services can be activated or scaled down based on requirements.
- Machine Learning Platforms: Instruments like TensorFlow and scikit-learn include built-in algorithms and workflows for training predictive models. They are frequently used to power tasks like forecasting, classification, and clustering.
The instruments employed often depend on the nature of the questions being asked, the size and complexity of the data at hand, and the team's familiarity with them.
Real-World Uses of Data Analytics
Data Analytics now occupies a central position across a wide array of industries. Consider how different sectors are putting it to use:
- E-commerce: Online retailers depend on data analytics to fine-tune pricing, detect fraud, track inventory, and deliver more relevant product suggestions. By observing how users browse and purchase, they can conduct more effective campaigns and boost conversions.
- Healthcare: Medical institutions are turning to data to predict health risks, guide treatment decisions, and manage resources more efficiently. Analyzing medical records and research data can lead to more accurate diagnoses and improved patient care.
- Finance: Banks and financial firms apply analytics to detect fraud, handle risk, automate trades, and improve support. Tracking real-time transactions and market trends helps them stay ahead of financial threats and act on opportunities.
- Manufacturing: In manufacturing, data supports smarter quality checks, maintenance planning, and production scheduling. By studying sensor data from equipment, teams can spot issues before they escalate and increase output efficiency.
- Transportation: This sector uses data analytics for route planning, traffic control, supply chain tracking, and autonomous systems. With the correct understandings, it's simpler to avoid delays, decrease idle time, and improve safety.
- Marketing: Marketing teams depend on Analytics to evaluate campaign results, segment audiences, personalize messages, and refine spending. Reviewing how users interact with campaigns helps marketers develop better strategies.
- Sports: Sports teams examine performance statistics to improve game plans, prevent injuries, and engage fans. Data assists coaches and analysts in making better decisions during and between matches.
These applications illustrate how Analytics is incorporated into actual business decisions across industries, turning data into a practical asset for daily operations and strategic growth.
Trends and Future of Data Analytics
As both business and technology progress, the methods teams use to work with data are also evolving. Here’s what’s driving change in the Analytics domain:
- Artificial Intelligence (AI) and Machine Learning (ML): AI and ML now play a larger role in routine Analytics, accelerating workflows and yielding deeper understandings. AutoML, in particular, simplifies the training and application of models without extensive manual coding.
- Big Data and Cloud Computing: The surge in large-scale data, combined with cloud-first strategies, is altering how companies address storage and computing needs. The cloud offers flexibility and helps teams work with massive volumes of data without excessive on-site resource development.
- Edge Computing: Processing data at or near its origin—rather than sending everything to the cloud—has become significant in contexts like IoT and self-driving vehicles. This shift helps shorten response times and manage latency effectively.
- Data Visualization and Storytelling: Communicating the insights derived from data is as important as performing the analysis. Interactive dashboards and concise narratives make it easier for non-technical audiences to act on data without needing to examine intricate details.
- Data Governance and Ethics: With data becoming more sensitive and central to business decisions, teams are now paying closer attention to ownership, privacy, and responsible usage. These discussions are occurring at both technical and leadership levels.
- Augmented Analytics: This approach introduces AI to assist with various stages, from data preparation to insight generation. It lowers the barrier for business users who require insights but lack deep technical expertise.
- Real-Time Analytics: As expectations for speed increase, there’s a greater focus on obtaining insights as events happen. Streaming data instruments and event-driven systems are becoming more widely adopted to support immediate decision-making.
All indicators suggest a future where Analytics becomes more deeply embedded in daily operations—accessible to a wider range of individuals, closely connected to business workflows, and a stronger catalyst for innovation and growth.
How Entrans Can Assist You in Utilizing Data Analytics
At Entrans, our focus is on helping businesses extract greater value from their data—regardless of whether you are just beginning or seeking to expand your capabilities. Here’s how we collaborate with clients:
- Data Strategy and Consulting: We work together with your team to develop a clear Analytics plan, based on your business objectives. This includes establishing sound data practices, navigating architecture decisions, and advising on the selection of appropriate instruments.
- Data Engineering: Our engineering teams construct the pipelines that connect all your data sources and prepare them for analysis. We make sure these systems are resilient and support future expansion.
- Data Analysis and Visualization: We undertake the complex work of examining data in detail, identifying trends, and creating visuals that effectively communicate the findings. From in-depth explorations to executive-level summaries, we help you understand the significance of the information.
- Predictive Analytics and Machine Learning: Our ML specialists develop models that assist in forecasting future events—whether related to customer behavior, equipment malfunctions, or operational hazards—so your teams can act proactively and with greater impact.
Want to know more? Why not book a free consultation call!
Data Analytics FAQs
What is the function of Data Analytics?
Data Analytics serves to extract meaning from unprocessed data and apply it to decision-making. This encompasses all stages from data gathering and preparation to conducting the analysis and ultimately drawing conclusions that support business objectives. It assists teams in identifying patterns, recognizing trends, and making choices based on evidence.
What analytical instruments are employed in Data Analytics?
The instruments you use in Analytics depend on the task at hand. For simpler tasks, spreadsheets like Excel or Google Sheets might suffice. For database operations, SQL is essential. When the analysis becomes more advanced, instruments like Python, R, Tableau, Power BI, Hadoop, or Spark often become necessary. Each instrument has its specific application depending on the scale and nature of the problem you are trying to solve.
Will AI supplant the roles of data analysts?
AI is altering the way Data Analytics is performed, but this does not imply a complete replacement of analysts. While automation can accelerate basic tasks—such as cleaning datasets or generating standard reports—analysts remain necessary to interpret results, formulate pertinent questions, and apply findings to real-world challenges. The role is evolving, not disappearing.
Does Data Analytics necessitate coding skills?
This depends on the depth of analysis you are undertaking. If you are generating basic reports or constructing dashboards, you can manage without writing code. However, if you are working on intricate models, deep analysis, or machine learning, you will likely need to use instruments like Python or R. While coding is not a prerequisite for every analyst, acquiring these skills can unlock a wider range of capabilities.
Stay ahead with our IT Insights
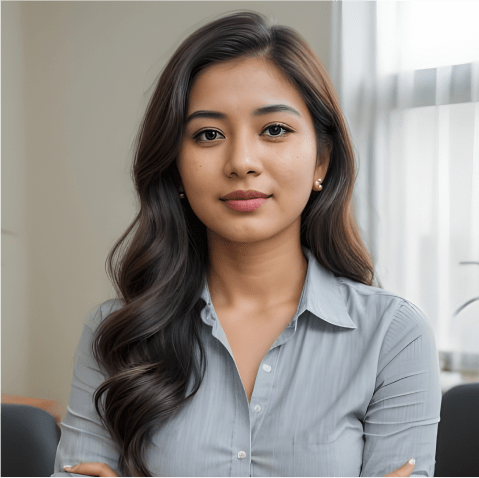
Discover Your AI Agent Now!
An AI Agent Saved a SaaS Company 40 Hours in a Week!