How Generative AI is Transforming the Product Lifecycle in Tech Industries
Generative AI is fundamentally changing how products are conceived, developed, and managed. Industry giants are driving this trend, and here’s what you need to know in order not to miss out!
Key Takeaways:
- Generative AI in Tech Industries can dramatically speed up your GTM in all stages.
- Using it can be a challenge due to your existing tools but it is needed and adds a lot of value.
- In using it you need to keep in mind data compliance laws, legal implications, and shadow IT. Not doing so can easily lead to costly fines and lawsuits. Which is why working with seasoned AI engineers is important.
What is Generative AI?
Generative AI refers to systems that can create new content or solutions by learning from existing data. These AI models produce text, code, images, or even synthetic data based on user inputs or defined rules.
By mimicking human creativity, they enable faster problem-solving and innovation in software development and product management industries.
Stages of Product Lifecycle in Tech Industries
- Idea Generation and Market Research: This stage involves brainstorming innovative ideas and conducting research to identify customer needs and market gaps.
- Design and Development: This is the stage where ideas turn into plans. Teams brainstorm, research, and create blueprints for the product. The goal is to figure out how it will work and meet users' needs.
- Prototyping and Testing: Next, early versions of the product are built. These prototypes are tested to find and fix any problems. Feedback during this phase helps improve the product before it’s ready for the market.
- Product Launch: This is when the product is introduced to the public. Marketing campaigns create excitement, and sales teams work to get it into the hands of customers
- Product Iteration Based on Feedback and User Experience: User feedback is collected after the launch. Improvements and updates are made to fix issues, add features, or improve performance based on what customers want.
- Scaling and Expanding Reach: If the product is successful, efforts focus on reaching more customers. This might mean selling in new markets, boosting production, or partnering with other businesses.
- Optimizing Operations to Compete With Competition and Improve Profitability: As competitors emerge, businesses find ways to stay ahead. They look for ways to lower costs, speed up delivery, or improve quality to make the product more profitable.
- Product Decline: Over time, demand for the product may drop due to newer options or changes in the market. At this stage, companies decide whether to update the product, shift focus, or phase it out completely.
How Generative AI is Transforming Each Stage of the Product Lifecycle
Gathering and Documentation of Requirements
Generative AI makes it easier to gather and document requirements by analyzing past projects, customer data, and industry trends. It helps teams avoid missing key details and saves time by organizing insights into actionable points.
With AI, teams can anticipate user needs better, making product development more focused and user-friendly.
- Use natural language processing (NLP) tools to understand requirements.
- Verify outputs with stakeholders.
- Keep requirements documentation clear and accessible.
Planning Your Project Timeline and Cost Estimation
Generative AI provides detailed project timelines and cost estimates by studying historical data and resource patterns. It prevents surprises like unexpected delays or cost spikes, giving teams more control.
AI also helps decision-makers allocate resources effectively, ensuring smoother progress and predictable outcomes.
Generation of Synthetic Test Data During Prototyping and Testing
AI generates realistic test data that mimics real-world scenarios, allowing teams to test thoroughly without depending on actual samples. This speeds up testing, identifies potential issues earlier, and reduces risks.
Synthetic data also makes simulations more flexible and cost-efficient, empowering teams to experiment confidently.
- Ensure synthetic data represents real-world conditions.
- Validate data to avoid false positives.
- Protect sensitive data during simulation.
Better Vulnerability and Smart Testing During Prototyping and Testing
Generative AI helps uncover weaknesses in prototypes and recommends solutions, leading to stronger and safer products.
It predicts potential problems before they escalate, saving time and resources. Teams can trust that AI-assisted testing creates more reliable results, reducing post-launch headaches.
Automated Code Generation and Bug Detection During Development
Generative AI supports developers by writing, reviewing, and debugging code faster. This minimizes repetitive tasks and allows developers to focus on solving complex problems.
By identifying bugs early, AI ensures cleaner and more efficient code, improving overall product quality and user satisfaction.
- Verify AI-generated code for consistency.
- Use AI tools compatible with version control systems.
- Continuously train AI with diverse programming languages.
Quicker Documentation During Product Launch
Generative AI speeds up the creation of user manuals, FAQs, and technical guides, ensuring these resources are ready on time.
Allowing users to shape content to different audiences, making it easier for new and potential users to understand and engage with the product. Clear and timely documentation enhances the user experience, building loyalty and trust.
Benefits of Generative AI in Product Lifecycle Management
Reduces Your Time-to-Market
Generative AI speeds up every phase of product development, from planning to launch. It analyzes data quickly, automates repetitive tasks, and identifies potential roadblocks before they become delays.
This allows companies to respond faster to market changes, gaining a competitive edge and meeting customer needs more effectively. By minimizing bottlenecks, teams can focus on innovation and timely delivery
Automates Repetitive Tasks
Generative AI takes over time-consuming activities like data entry, documentation, and standard reporting. This allows team members to dedicate their energy to more strategic and creative work.
Automating mundane tasks reduces errors and improves accuracy, while also helping to prevent burnout among employees. With AI handling the groundwork, teams can operate at a higher level of efficiency.
- Clearly define automation boundaries.
- Regularly evaluate task relevance.
- Maintain system reliability to avoid disruptions.
Lowers Operational Costs
By streamlining processes and reducing manual workload, AI helps companies save money. It optimizes the use of resources such as time, labor, and materials, allowing businesses to achieve better results with less effort.
The cost savings can be reinvested into innovation, new tools, or enhancing the user experience, creating a virtuous cycle of improvement without sacrificing quality.
Accelerates Design Iterations
With generative AI, product designers can test and refine ideas more rapidly. AI models generate multiple variations of a design, providing valuable options to evaluate. This leads to faster alignment with user needs and market trends.
Quick iterations mean products are fine-tuned to perfection before they even hit the market, resulting in higher customer satisfaction.
- Update AI systems with user feedback.
- Avoid over-iterating on designs.
- Test changes in controlled environments.
Improves Decision-Making with Predictive Insights
Generative AI uses patterns in data to forecast trends, risks, and opportunities. This empowers teams to make proactive, well-informed decisions throughout the product lifecycle.
By combining AI insights with human expertise, businesses can optimize strategies, reduce uncertainty, and drive better outcomes. Predictive analytics ensures resources are allocated efficiently and goals are met effectively.
Challenges and Risks of Gen AI in Product Development and Lifecycle Management
Accuracy Limitations Due to Absence of Data
AI relies on high-quality, up-to-date data. Without this, its predictions and suggestions can miss the mark. For designers and companies, this could mean products that don't meet user needs, leading to frustration and a poor user experience.
What can you do? Regularly refresh datasets to reflect current trends, gather insights from diverse sources to avoid bias, and always compare AI outputs to established benchmarks. This ensures your tools stay relevant and trustworthy.
Lack of IT Talent
AI systems require skilled hands. If your team doesn't have the expertise, it could slow down progress or lead to expensive mistakes in the long-run. This impacts the GTM timeline and stakeholders who rely on smooth workflows and companies aiming to stay competitive.
To deal with this, you’ll likely need to create training opportunities for your current team, bring in external specialists to fill gaps, and choose AI tools designed with simplicity in mind so anyone can use them effectively.
Operational Disruption
Bringing AI into your processes can shake things up. Employees might feel overwhelmed, and workflows could slow down. For product stakeholders, this means delays in testing and iterating ideas, and for companies, it means a longer go-to-market timeline.
How to manage: Break the process into small steps. Let everyone know how AI will improve their work, and provide hands-on training to ease the transition. This keeps teams confident and workflows steady.
Incompatibility With Existing Systems or Legacy Systems
Generative AI has the potential to revolutionize product lifecycle management, but compatibility issues with legacy systems remain a significant challenge. Legacy systems often lack the necessary flexibility and architecture to support advanced AI models, hindering their ability to fully leverage AI-driven insights. T
his leads to inefficiencies, as AI tools may struggle to operate with older data formats or incompatible software components.
Legal and Ethical Issues
Since generative AI can be applied across the product lifecycle, managing legal and ethical challenges can be difficult due to varying laws and guidelines. With the right AI framework, you can standardize decision-making processes and ensure consistency across all stages.
But to deal with this, you'll need to:
- Stay informed about AI regulations.
- Establish transparent usage policies.
- Conduct regular audits for compliance.
Privacy and Creation of Shadow IT
Since generative AI can be applied across the product lifecycle, handling privacy concerns and the risk of shadow IT can be difficult due to varying data protection regulations. With a solid AI framework, you can centralize data access and control.
More importantly, you can also reduce the risk of shadow IT by implementing clear data governance policies across all product stages. Also encrypting data and setting company-wide policies can keep this aspect in check.
Future of Generative AI in Tech Industries
Even Easier Development Processes for Software Developers
Generative AI is reshaping software development by creating reusable, adaptable code across languages and frameworks. Developers can expedite workflows using AI-generated snippets, reducing repetitive tasks while maintaining precision.
Generative AI will further simplify coding, making software development more accessible to non-experts.
Additionally, AI-driven tools simplify complex coding challenges by enabling automated debugging and context-aware recommendations, improving developer efficiency.
More Industry-Specific Generative Apps for All Professions
By processing specialized data, generative models create more relevant outputs, from design to decision-making.
This evolution allows professionals to access smarter tools that adapt to their field's nuances, improving productivity and delivering deeper insights without requiring significant manual oversight.
Better Customer Segmentation for Intelligent Marketing
As generative AI continues to evolve, it will change and is already changing customer segmentation by analyzing large datasets from various sources.
This enables the identification of patterns and trends that human analysts might miss. By using AI to process diverse data points, marketing strategies become more targeted, helping businesses reach specific customer segments with greater precision.
But in doing this, users need to keep in mind compliance practices like:
- Use anonymized data for segmentation.
- Regularly update segmentation models.
- Align marketing strategies with user expectations.
More Diverse Data From Various Data Sources
As generative AI continues to evolve, it will rely more on diverse data from multiple sources to improve decision-making and innovation within tech industries. With access to real-time data, machine learning models can produce more accurate insights and predictions.
Access to diverse datasets will improve AI accuracy and relevance, ensuring better decision-making and innovation.
How Entrans Can Help You Leverage Generative AI for Product Lifecycle Transformation
Entrans speeds up your GTM with efficient workflows, and lower costs accelerating your time-to-market.
Simplify how generative AI across the product lifecycle, offering solutions that enhance design processes, streamline production, and optimize end-to-end workflows. Our expertise ensures seamless collaboration across teams while addressing regulatory and ethical challenges.
Want to transform your product lifecycle with generative AI? Reach out for a free consultation!
Stay ahead with our IT Insights
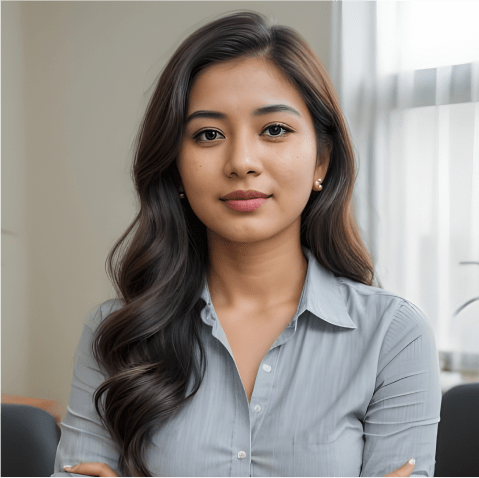
Discover Your AI Agent Now!
An AI Agent Saved a SaaS Company 40 Hours in a Week!